Agentic AI: Capabilities and Potential Applications
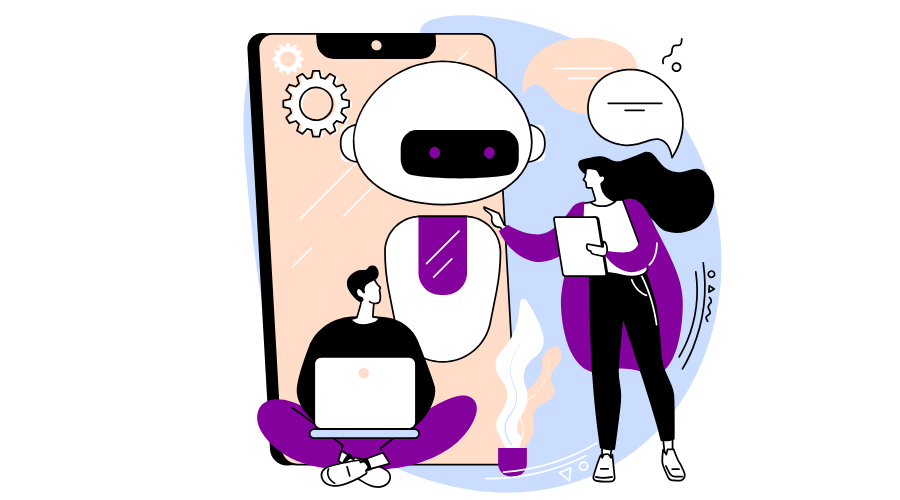
Artificial intelligence (AI) has advanced remarkably since its inception. It has now moved from rule-based systems to complex machine leaning models.
Currently, we sit at the brink of a new era led by the surfacing of agentic AI. It’s the ultimate paradigm shift that promotes AI systems with independence in decision-making and problem-solving capabilities.
Agentic AI is the true representation of central advancement in the industry of AI, providing the possibility to make far-reaching changes in different fields and re-structure the science of human-machine interaction.
The article dives deep into the prominent areas where agentic AI is to have a part in making considerable impact including but not limited to IT Operations, Human Resources (HR), Customer Service, Sales, Manufacturing, and Finance & Fraud monitoring.
We zero in on portraying the evolutionary potential of agentic AI and its impact on the future.
Definition of Agentic AI
Agentic AI is a fast-emerging concept referring to Artificial intelligence systems that have a level of autonomy.
What does this mean? It can make independent decisions unlike traditional AI, which is mainly reactive and goal oriented. Agentic AI takes it up a notch by being proactive, learning from past mistakes and experiences, and being flexible enough to adapt to evolving environments.
Key differences between Agentic AI and Traditional AI
Feature | Traditional AI | Agentic AI |
Autonomy | Autonomy is restricted, human intervention is required | Hugh level of autonomy, making it capable of independent decision making |
Learning | Acts on predefined rules and data | Adapts easily to new information by learning from experiences |
Goal-oriented | Prioritizes specific tasks and gaols | Seeks opportunities and tackles challenges by being proactive |
Behavior | Responds to stimuli | Initiates actions and plans future steps |
Innovations enabling Agentic AI
There are many technological advancements that have given way for the progress of agentic AI:
1. Large Language Models
Models such as GPT-3 and LaMDA are game-changers for Natural Language processing. They are like the pioneers in enabling AI systems to understand, generate and be responsive to human language in a polished manner.
2. Scalable Computing Power
The never-ending availability of dominant computing hardware including GPUs and TPUs has enabled the training and development of complex AI models.
3. Massive Datasets
Data augmentation generated by different sources helps AI systems with the necessary elements to learn and improve as well.
Key Capabilities of Agentic AI
Crowned AI researchers Yann LeCun and Geoffrey Hinton have made groundbreaking contributions in the field of agentic AI.
They have laid the foundation for development of agentic AI through deep learning and neural networks establishing more intelligent and autonomous systems.
Autonomy: The Bedrock of Agentic AI
Agentic Ai systems function independently requiring minimal human intervention. This is truly visible in AI-enhanced virtual assistants out there like OpenAI’s GPT-based models.
They can independently generate ideas, content, recommendations and help in making decisions with the user constantly refining inputs.
Agentic AI’s capacity to act autonomously makes these systems invaluable in key areas like education and real-time business analytics.
Reasoning and Decision-Making: The Cognitive Core
Agentic AI surpasses the ability to make context-based judgments, deploy strategic management and even balance trade-offs.
For example, systems like IBM Watson show outstanding reasoning by diagnosing medical conditions with the help of contextual data analysis.
Healthcare prioritizes such applications of Agentic AI because of this capability where quick and accurate decision-making is crucial.
Adaptable Planning: Navigating Dynamic Environments
Adjusting plans to evolving scenarios makes Agentic AI stand out from traditional AI. It is known to thrive in dynamic environments without fail.
For instance, autonomous vehicles continuously interpret sensors to data drive through impossible traffic conditions, this done by employing adaptable planning.
Systems remain very effective even during cases of unexpected challenges because of Agentic Ai’s adaptable planning.
Language Understanding: Bridging the Human-Mahine Divide
Agentic AI uses Natural language processing (NLP) to understand and deliver on complex instructions.
For example, NLP allows OpenAI models to seamlessly integrate and facilitate interactions across different industries.
NLPs are the reason why important business elements like customer service and content creation can be enhanced by understanding user intent clearly and responding appropriately.
Workflow Optimization: Streamlining Processes and Boosting Efficiency
A very important ability of agentic AI lies with streamlining workflows. It does this by seamlessly switching between tasks and applications without any interruptions.
In an enterprise environment, UiPath’s robotic process automation (RPA) allows optimization of workflows by:
- Automating repetitive tasks
- Enhancing productivity
- Reducing operational costs
The capabilities of Agentic AI is key to achieving positive and progressive impact across different industries. But their implementation and development is to be handled with careful consideration.
Technical Architecture of Agentic AI
Agentic AI exhibits a complex stage of evolution in artificial intelligence. It is based on three core components: perception, cognition and action.
The triad makes sure an effective workflow is maintained at all times right from data acquisition to decision-making and implementation.
Core Components of Agentic AI
Perception Layer
The perception Layer of Agentic AI is responsible for collection, organizing and interpreting data picked up from the environment.
Sensors, cameras or input systems are used to collect the data. AI recognizes patterns and responds accurately due to its ability to contextualize the processed data.
Cognition Layer
Natural Networks and decision trees are advanced algorithms that help Agentic AI to understand context and enable decision making by processing the interpreted data.
Action Layer
Actuators and output mechanisms help execute decisions. They help facilitate a smooth interaction between the AI and its environment, thus executing the completion of autonomy.
Principles of Design
Modularity
Modularity is the reason why perception modules and reasoning engines can function autonomously while executing a seamless integration.
It ensures the individuality of Agentic AI. The principle can expedite scalability and upgrades without interrupting the entire system’s workflow.
Scalability
Agentic AI can adapt to increasing data volumes and complexity with the help of the Scalability principle.
The systems powerfully allocate computational power so as to not underperform as demand grows. It does this by using distributed computing and cloud-based resources.
Interoperability
Interoperability is very important for enabling teamwork between its modules and external systems.
Standardized protocols and frameworks make sure platforms and devices achieve compatibility through standardized protocols and frameworks.
Adaptability
All the systems respond powerfully to evolving and uncertain environments. Agentic AI fine-tunes its responses to meet complex challenges through real-time feedback mechanisms and reinforcement learning.
Single-Agent vs Multi-Agent Systems
Single-agent systems are way easier to design and manage. But since they are so simple and centralized they might struggle with adaptability and subsequent scalability.
Whereas Multi-agent systems have many specialized agents working together as a team to provide resilience, scalability and promoting decision-making for compounded tasks.
Thus, agentic AI provides fluidity and ground-breaking solutions across various industries, right from automating daily routine tasks to pitching strategic management at scale all with the help of these architectural principles and using advanced technologies.
Potential Applications of Agentic AI
Traditional workflows are being replaced by agentic AI since it is more efficient, precise and innovative in nature.
Agentic AI’s applications cover a wide range of industries allowing businesses to optimize operation, save time and enhance productivity.
Here are some major areas where agentic AI has made a significant change:
IT Operations
Agentic AI is playing its own league in IT departments leading automation of mundane (but critical) tasks. This includes handling routine system requests and performing system maintenance as well.
For example, you can use AI-driven systems such as AIOps (Artificial Intelligence for IT Operations) to oversee and manage IT environments continuously, predict failures, suggest fixes and detect issues proactively.
Moogsoft uses AIOps to detect anomalies and reduce alert fatigue to promote faster resolution times.
Reliability is increased and downtime is decreased using such solutions, agentic AI capabilities prove to be immeasurable.
Dynatrace and AppDynamics are also two other agentic AI-driven solutions that help perform monitoring and make sure IT teams check system reliability off their lists.
Agentic AI not only helps in programming but it also takes strides in debugging as well. It uses machine learning algorithms to recognize and tackle coding errors efficiently.
Thus, streamlining makes sure deployments are faster and software solutions are stronger than ever.
Human Resources (HR)
Running a business has its own administrative burdens; agentic AI untangles these difficulties and helps departments operate efficiently.
For instance, Workday helps in repetitive processes including payroll management, benefits administration, and recruitment which is easily automated using agentic AI.
There’s also HireVue that can screen resumes, set-up interviews and in extreme cases even conduct candidate assessments without any error. This saves up so much time for HR teams,
Additionally, agentic AI systems like Oracle HCM Cloud and SAP SuccessFactors offer insights into workforce planning by analyzing trends in employee engagement and performance.
Hence organizations can now make data-driven decisions about talent management and retention strategies.
Customer Service
The collaboration between customer service and Agentic AI has restructured how businesses engage with their customers on a daily basis.
NLP (Natural Language processing) assisting virtual agents and chatbots like Ada are available 24×7 to answer customer queries without any human intervention.
Zendesk AI is another agentic AI-powered chatbots that effortlessly handles routine queries and escalating complex issues to humans whenever needed. This reduces response times significantly too.
Additionally, Genesys AI helps tailors customer interactions by analyzing data, this is done to recommend products and services based on customer’s individual preferences.
This approach improves user satisfaction and customers keep coming back.
Sales
Agentic AI has reinvented the whole fundamentals of sales processes by automating lead management, customer outreach and forecasting
Tools like HubSpot Sales Hub use agentic AI to get leads, allowing the sales team to focus on chasing high-quality prospects while automating subsequent follow-ups.
Such AI-powered solutions such as Gong also help analyze interactions with the customers and offer actionable insights into communication that can help boost conversions.
Additionally, recommendation engines also improve customer engagement using agentic AI by providing personalized suggestions. This is done by analyzing purchase and browsing behavior.
For example, Salesforce Einstein integrates AI to give insights using predictive analytics. It guides salespeople to build the next best actions.
Agentic AI empowers the sales team and keeps their morale up by helping them make data-driven decisions and close deals faster.
Manufacturing
Agentic AI has seeped into manufacturing too, the field has benefitted a lot especially in workflow optimization and maintenance predictions.
Solutions such as Seebo and GE Predix accelerate efficiency by overseeing production lines in real time and recognizing bottlenecks easily.
IBM Maximo also helps analyze sensor data so that equipment failures can be detected without any issue decreasing unplanned downtime and repair costs.
Quality assurance is not left behind, agentic AI takes care of that too. NVIDIA Clara Guardian is a huge hack that uses machine learning to detect defects and make sure product standards are consistent.
Hence, manufactures can also improve reliability, product quality and cut costs by using Agentic AI.
Finance & Fraud Monitoring
Even the financial sector is not a stranger to agentic AI, it has become a necessity in the industry to detect fraud, manage compliance and financial auditing as well.
Feedzai and Fraud.net are two great tools to monitor transactions in real time, they help in recognizing unusual patterns and prevent fraud subsequently.
Machine learning algorithms, one of agentic AI’s weapons, is used to make sure the business is well-protected against new techniques of financial fraud.
For Compliance and audits too, AppZen is a great tool to automate document analysis, and point out inconsistencies. It also offers actionable insights into regulatory compliances.
Also, KPMG Ignite integrates agentic AI seamlessly into financial reporting and auditing processes that helps reduce manual workloads and enhance accuracy.
Thus, there are many solutions out there that promote agentic AI as a growing application in complex and important fields such as finance too.
Agentic AI for Product Managers
Agentic AI owing its strength to autonomy, adaptability and decision-making abilities has now changed how AI operates across different industries.
It’s a huge powerhouse for product managers who aim to succeed in their field. It provides ever-changing tools to tackle issues in collaboration, workflow optimization and decision making.
Enhanced Decision-Making with Data-Driven Insights
Agentic AI systems handle huge volumes of data simultaneously. It understands data and provides actionable insights that help product managers make quick and effective decisions.
OpenAI’s GPT models and Google’s DeepMind are great tools to analyze historical trends, competitive landscapes and even consumer behavior as well. Forecasting outcomes and optimizing product strategies become a piece of cake.
The insights help product managers with assessing risk, anticipating market shifts and aligning product features with business goals as well.
For instance, platforms like Amplitude, an AI-driven platform, uses data analytics to point out recommendations and feature improvements. This helps the product team focus on improvements easily.
Workflow Automation for Strategic Focus
A product life cycle includes lots of repetitive tasks such as data entry, reporting and performance tracking.
Agentic AI frees up time for product managers to execute strategic planning and innovation by automating these workflows.
Jira and Trello can be effortlessly integrated with tools like Zapier to enhance project management.
This way, routine tasks such as project status updates and report generation can be automated and product managers can solely focus on stakeholder alignment while decreasing operational overhead.
Proactive Problem-Solving and Market Adaptability
Agentic AI holds the superpower to recognize problems even before they surface. It excels at using predictive analytics; Agentic AI predicts challenges in customer, adoption, market demand as well as supply chains.
For example, Salesforce Einstein AI analyzes engagement data to anticipate customer churn risks. It helps product managers to execute proactive retention strategies.
Hence, product managers can respond quickly to uncertain environments making sure that the product remains competitive in any condition.
Enabling Better Team Collaboration
Consistent communication and collaboration are part and parcel of successful product management.
Agentic AI facilitates collaboration using:
- Data Centralization
- Comprehensive updates generation
- Stakeholder alignment
Notion AI is a great AI-driven tool to sum-up meeting notes and track deliverables along with task prioritization suggestions.
Customization and Scalability for Growing Portfolios
Managing a diverse pool of offerings becomes more and more difficult as your product portfolio keeps scaling.
Agentic AI helps you simplify this by providing personalized recommendations and effective resource allocation.
There are many tools available like Adobe Sensei that provide custom insights making sure every product’s demands are met efficiently.
Additionally, scalable AI can easily tackle growing data needs by accommodating and helping product managers to scale operations without any difficulties.
Thus, the mix of intelligence, autonomy and adaptability makes Agentic AI an unbreakable ally for modern day’s product managers.
Challenges and Best Practices for Implementing Agentic AI
Agentic AI is an innovative addition to the field of artificial intelligence that focuses on independence in decision-making, hence leading systems to undertake very difficult tasks with less human intervention.
But, depending on such complex systems comes with its own sets of challenges and obligations to best practices necessary for ethical, efficient and safe operations.
Key Challenges
Flawed Recommendations:
The biggest challenge in agentic AI comes with its dependence on complex algorithms that can produce inaccurate or biased results at times.
Even though Agentic AI promises minimal human intervention, without proper oversight, the slightest of errors can have consequences in real-world scenarios.
Complexity and Transparency:
Agentic AI is an expert at decision-making; true, tracing back to how it arrives at a particular decision is very difficult because of opaque algorithms.
Thus, lack of transparency can cause mistrust among users and said decision makers.
Ethical Concerns:
Ethical issues have always been a problem when it comes to using AI, especially when it comes to giving autonomy to artificial intelligence.
There are many issues including but not limited to the need for accountability in case of errors and harmful decisions, concerns about data privacy and misuse, etc.
Security Risks
Agentic AI is known for largely interacting with diverse datasets; this increases the risk of data breaches and accessing unauthorized data.
The flexible nature of these systems make them obviously susceptible to security sensitivity.
Compliance and Regulation
Regulatory legalities keep changing with the dynamic nature of compliance measures, keeping up with these landscapes is a strenuous task.
Non-compliance to these measures, especially in the context of handling agentic AI can lead to legal and financial consequences, thus undermining trust in technology.
Best Practices for Implementation
Human Oversight and Transparency
Keeping an actual human being in the loop while handling agentic AI can be one of the best approaches to make sure that critical decisions are overseen and can be withdrawn when necessary.
Deploying explainable AI techniques to illuminate how decisions are taken develops trust and helps in accountability.
Audits for training data and system processes also improves transparency.
Rigorous Testing and Error Handling
Before releasing agentic AI, it is important to identify and avoid flaws in decision-making processes, hence extensive testing becomes necessary here.
Error-catching mechanisms and real-time monitoring can help tackle issues proactively reducing risks of erroneous outcomes.
Governance Frameworks
A durable governance policy makes sure AI is used responsibly.
Creating protocols for every step including but not limited to decision-making, user content and data handling is a great way to stay compliant with industry regulations and standards.
Ethical Considerations
Implementing ethical principles into the design, deployment and execution phase is crucial. Everyone working towards the success of Agentic AI must deploy guidelines.
These guidelines can include anything from ensuring fairness, reducing biases to safeguarding user data and making sure boundaries are set to prevent data breach.
Regularly coming back to these principles can help adapt to changing societal and technological nuances.
Strengthening Security Measures
Not waiting for problems to arrive and proactively confronting security vulnerabilities is crucial. This includes encrypting sensitive data, controlling access management, and establishing response plans to avoid breaches.
System integrity and user privacy are upheld this way when with regular security checks and assessments.
Future Directions of Agentic AI
Agentic AI is revolutionizing the way industries will function by allowing machines to independently rake over complex tasks and consolidate itself with future technologies.
Let’s look at some important trends that will highlight the importance of Agentic AI in the coming future:
Emergence of AI Copilots and Virtual Employees
Agentic AI is developing into the supportive co-worker everyone needs, achieving great heights by assisting with decision-making and task execution.
AI copilots are very useful in decreasing workload, especially in areas of repetitive tasks.This includes HR onboarding, customer service, and even vigorous decision-making fields such as healthcare and finance.
For example, these AI systems can not easily recognize and flag illegal activities in banking and flaws in recommended treatment plans in healthcare. It adapts to changing scenarios in real-time.
Hence, AI’s role as a virtual employee not only decreases operational burden but also helps humans focus on creative and strategic elements of work.
Integration with IoT, Blockchain, and Low-code / No-Code Platforms
Agentic AI prospers when it is unified with complimentary technologies. It can collect and organize real-time data from anywhere using IoT.
This promotes informed decision making in areas like supply management and most importantly, smart cities.
Blockchain integration also improves the security and transparency of every AI-driven system, making sure trust is upheld in autonomous decision-making.
Plus, agentic AI allows non-technical users to build solutions when integrated with low-code / no-code platforms accelerating AI capabilities.
Such consolidation is crucial to expanding the accessibility and scalability of AI.
Advancing Human-AI Collaboration
Agentic AI future gives way to complement human intelligence instead of replacing it completely. The partnership is quite visible in the increasing adoption of AI copilots providing decision support and mundane task handling.
It leaves humans free to focus more on important tasks. Not only mundane tasks but even complex procedures such as strategic decision-making processes are being augmented by agentic AI.
Agentic AI provides real-time insights and recommendations that help executives in surfing complex business challenges.
The Road Ahead
Businesses should focus on targeting opportunities where agentic AI systems can add utmost value if they want to harness its power completely.
Agentic AI should be integrated in every existing workflow and make sure they are designed in such a way that teams can collaborate with it easily.
By doing this, organizations can open futuristic advantages. Agentic AI is centered to restructure the limits between what a machine and a human being can achieve if they team up.
Conclusion
Agentic AI is a revolutionary success enabling positive transformation across all industries. It cannot be stressed enough that its applications are useful everywhere.
The capabilities of Agentic AI not only improve process and saves up time but also opens up new avenues for innovation along the way directly as well as indirectly.
In product management, the long-term implications of agentic AI are very important. It helps product managers and teams to make data-driven decisions through all-round collaboration.
In spite of all the positive aspects, agentic AI, with great potential comes the greater responsibility of maintaining ethical implementation.
Finding balance between automation and human oversight is very important for developing trust and accountability as well.
A transparent algorithm, strong compliance, and continuous human involvement is the correct way to avoid risk and maximize benefits.
To conclude, agentic AI is not just for automating processes and improving efficiency, when used correctly it can prove to be a useful partner for innovation. Thus, the future rests within the synergy between agentic AI, human creativity and judgment.