There Is More to AI Than Just Gen AI – Chisel Product Talks
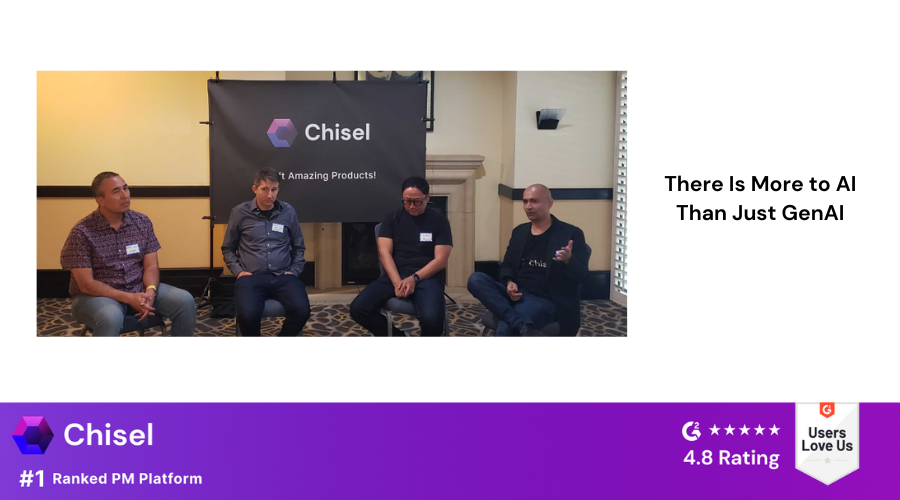
This discussion includes,
- Introduction and Overview of AI Applications
- Transforming Manufacturing Through AI and Computer Vision
- Revolutionizing Product Design and Automation with AI
- Exploring the Unique Challenges and Opportunities in AI Beyond GenAI
- Understanding AI in Physical and Digital Spaces: Insights from Industry Experts
- The Role of AI in Product and Investment Decisions
- Ensuring Accuracy and Safety in AI-Driven Systems: Lessons from the Field
- The Evolving Role of Product Management in an AI-Driven World
- Embracing AI While Staying Strategic
- The Human Touch in Design and Product Management: AI vs. Intuition
- Ethical Considerations and Risks of AI
Introduction and Overview of AI Applications
AI has taken the world by storm, with Generative AI (Gen AI) often at the forefront of the conversation. But there’s a lot more happening in the AI space than just Gen AI.
Today, we’re diving into the broader world of AI, exploring what leaders in the field are working on beyond the buzz of generative models.
From computer vision to performance management and even self-driving cars, AI’s potential stretches far and wide.
We’re joined by
Mark Hall, a former Meta product leader and now founder of a performance management startup
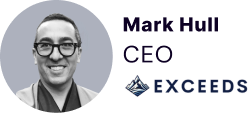
Chris Van Dyke, founder of Overview, which focuses on computer vision.
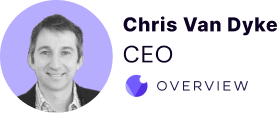
Jae Park, a senior product leader at Ford working on cutting-edge self-driving technology
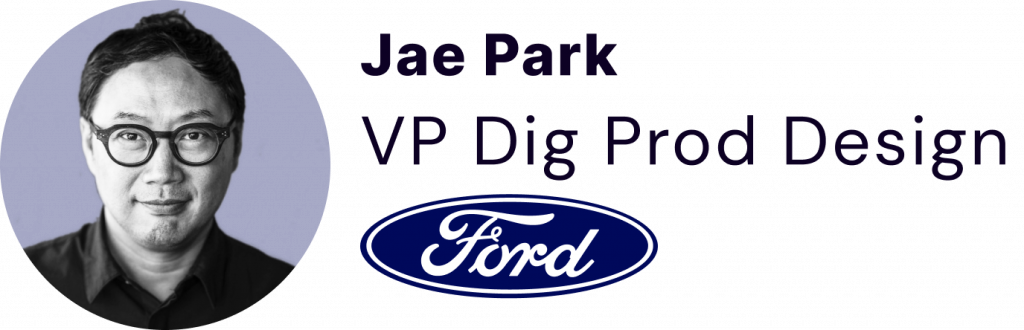
Praful, the genius behind Chisel, revolutionizing the way product managers work.
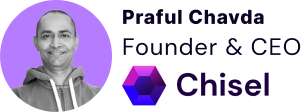
Each of them brings a unique perspective, showing that AI’s impact is being felt across a variety of industries.
So, let’s get into their experiences and learn how AI is shaping innovation in ways that go far beyond what we typically think of as “AI.”
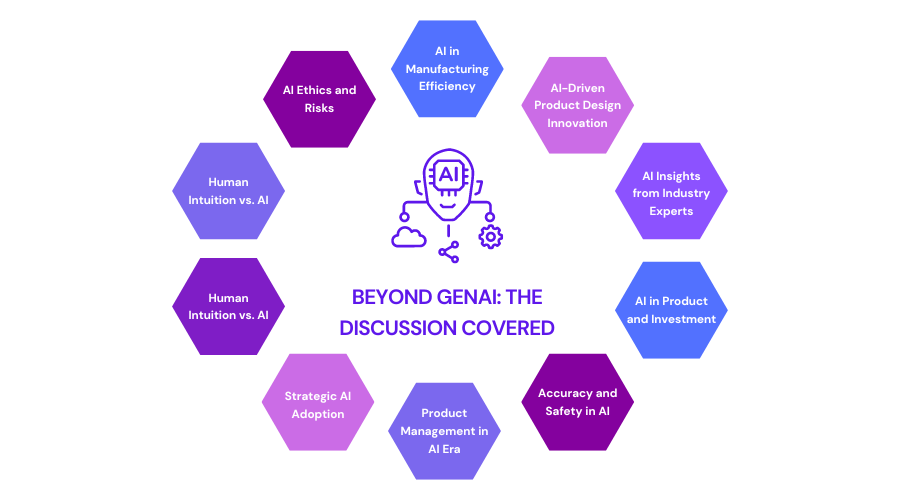
Transforming Manufacturing Through AI and Computer Vision
AI in manufacturing is making waves, especially when it comes to computer vision, which offers a powerful way to streamline processes and improve quality control.
Chris, the founder of Overview AI, has firsthand experience with this, having previously worked at Tesla in the manufacturing space.
Chris mentions I started a company in 2019, building an AI tool. Even then, computer vision was getting very good, so you could start to do applications that were challenging before.
He saw the potential of AI early on and started Overview in 2019, focusing on using computer vision to tackle some of the most challenging problems in manufacturing.
What makes this approach so innovative is its use of AI that’s a bit different from the buzz around generative models. Overview AI uses a camera equipped with software that’s installed directly in factories.
This AI tool can be quickly trained to identify defects in products, making it highly efficient for industries that deal with repetitive production.
Instead of relying on cloud-based solutions, the AI runs locally on the device, providing a more secure and reliable option for factories that handle sensitive data or need fast, on-site results.
While it may not be the trendy Gen AI everyone’s talking about, computer vision is a deep, real-world application of AI that’s transforming manufacturing.
From spotting defects with pinpoint accuracy to improving overall production efficiency, this type of AI is making a significant impact on how factories operate.
By embedding AI directly into devices, companies like Overview are showcasing how diverse and versatile the AI landscape can be, especially in industries where precision and security are paramount.
Revolutionizing Product Design and Automation with AI
AI is playing an increasingly central role in product design and automation, transforming how companies build, refine, and manage their products.
Take Jae’s experience in product design at Ford and his journey with AI to improve user experiences.
One of his earliest forays into AI was with the Windows Phone, where he collaborated with a Microsoft AI scientist to develop a predictive keyboard. This was before the era of large smartphone screens, so the challenge was creating an intuitive typing experience.
How did Jae go on to innovate this? He says We made the tap size bigger, which was novel at the time, and it did way better than the iPhone because I still have problems typing on iPhones today. And so that was my first entry into working with someone and designing an interaction model that was intelligent and helpful and useful.
By leveraging AI, they could predict the next letter users were likely to type and adjust the size of the keys, significantly improving accuracy. This predictive model outperformed even the iPhone keyboard at the time, demonstrating AI’s power in enhancing product usability.
AI in product design isn’t just about creating intelligent user interfaces. At Amazon, Jae worked on the Echo Show, where they aimed to make voice interaction more natural and fluid.
Although technological limitations at the time prevented the smoothness they envisioned, the integration of AI laid the groundwork for the future.
Jae proposes “Now with the LLMs, I think the input can be much more natural, so I think that’s kind of exciting.” The voice input is much more seamless, allowing users to interact with devices in ways that feel intuitive and efficient.
In the automotive industry, AI is not just reshaping how cars—or rather, “vehicles”—are designed but also how they operate.
With autonomous driving becoming more prevalent, there’s a focus on transforming the car’s interior into a “third space”—a place where people can work, relax, or engage in other activities while in transit.
So Jae concludes You’re seeing a lot of that happen in China already. And so we think about, you know, different modalities while you’re going somewhere, when you’re stationary, or when you’re doing some activities around the vehicle, cars. And so those are all the opportunities we have to orchestrate really interesting experiences.
Beyond the design aspect, automation is another area where AI is making significant strides. Take Chisel, a product management platform that leverages AI to automate various tasks. According to its founder, one of Chisel’s unique differentiators is its focus on automation.
Praful, Chisel’s proud founder continues. For instance, users can say ‘Hey, the June release just finished, so create a status report for the June release’. Then we will go through every feature or work item in your workspace, and automatically include that in the status report. So we do a lot of automation to free up your time.
In addition to automation, Chisel integrates discovery and roadmap features, providing complete traceability from customer feedback to final product implementation.
This is a game-changer for product managers who want to ensure that every feature is backed by data and aligned with customer needs.
The platform’s AI-driven approach enables more efficient product development and reduces the need for multiple tools.
In short, AI is transforming product design and automation in ways that go beyond the hype of generative AI.
Whether it’s creating more intuitive interfaces, enabling seamless voice interactions, or automating repetitive tasks, AI is making products smarter, more efficient, and more user-friendly. The future of AI in these fields is exciting, with endless opportunities.
Exploring the Unique Challenges and Opportunities in AI Beyond GenAI
In a conversation that delved deep into the realm of AI and its evolving landscape, Praful and Mark explored the intersections between traditional machine learning (ML) applications and the rapidly growing domain of Generative AI (GenAI).
They reflected on their vast experiences, from building products at Microsoft and Facebook to leading efforts in AI-driven HR technology, discussing what sets these areas apart from the GenAI buzz and the unique challenges product leaders face today.
Differentiating AI Applications Beyond GenAI
Praful, having extensive experience as Head of Product at companies like Humu and Microsoft, began by raising an essential question.
He noted how different AI applications are, particularly in areas outside the standard GenAI frameworks. “Each of us is working in an area where the AI application is very different from a typical GenAI application,” he pointed out.
This led to his core query: What makes these applications unique, and what distinguishes them from the growing popularity of GenAI?
Mark, who has a background at Facebook and is currently in a role where GenAI plays a more prominent part, responded by emphasizing both the appeal and the complexity of GenAI.
He acknowledged that while GenAI’s accessibility is a major advantage, making it easier for more people to experiment with AI, it also presents unique challenges: “It’s deceptively easy. It’s pretty difficult to get the right kind of signal, the high-quality output out of it, despite how easy it is.”
This ease of use might spark creativity, but achieving meaningful, high-quality results still requires deep expertise and understanding of the underlying systems.
Moving Beyond the Novelty of GenAI
Mark noted that while GenAI is drawing massive attention due to its accessibility and potential, much of the current excitement is focused on novelty rather than solving real-world problems.
As he put it, “We’re in this novelty phase where, honestly, I question a lot of the things that are out there, especially related to how we’re solving a real problem.”
This critique highlights a common concern among product leaders: the rush to adopt new technologies often overshadows a focus on addressing genuine customer needs.
However, Mark also highlighted that when AI is properly implemented—especially in areas beyond GenAI—it holds tremendous power.
Referring to his time at Facebook, he shared how AI was used to tune user experiences, predicting actions such as comments or posts: “We were tuning things all the time, trying to predict what will be the thing that leads somebody to comment, to post, to do whatever.”
This process, while once time-consuming and resource-intensive, is now made more efficient with today’s AI tools, enabling faster model generation and testing.
The Importance of Data Fundamentals
Despite the exciting advancements in AI, Mark stressed that there are still critical fundamentals that product teams must master—especially when it comes to handling data.
He warned of the dangers of leveraging GenAI without a deep understanding of data pipelines: “If you don’t understand the data fundamentals, how data comes in the pipeline, the way that you need to structure it, how to organize it, it’s easy to use those GenAI tools in a more dangerous kind of way.”
This statement underscores a key takeaway for any product leader working with AI today: while tools like GenAI are powerful, they require careful management and understanding to avoid potential pitfalls.
Simply adopting the latest trends won’t automatically lead to success—there’s still a significant need for strong foundational knowledge in data handling and AI systems.
As the conversation wrapped up, it became clear that while GenAI has introduced new possibilities for product leaders, the broader world of AI remains incredibly diverse, complex, and full of untapped potential.
Both Praful and Mark emphasized the importance of exploring these different avenues of AI—whether in HR tech, NLP, or more personalized predictive models—while maintaining a focus on real-world problems.
Product leaders must navigate the delicate balance between innovation and practicality, leveraging both cutting-edge AI tools like GenAI and more traditional ML approaches to build solutions that truly meet user needs.
As Mark aptly summarized, “Ultimately, we have to be building things that will solve real problems and meet real needs.”
Understanding AI in Physical and Digital Spaces: Insights from Industry Experts
The conversation between Chris, Jae, and Praful offers a deep dive into how artificial intelligence (AI) is used in both physical and digital realms, particularly focusing on the nuances of General AI (GenAI) and traditional AI in real-world applications.
These industry experts share their thoughts on the practical uses of AI, emphasizing that while GenAI is a powerful tool, it doesn’t always provide the best solutions, especially in highly specific or physical contexts.
Finding the Right AI for the Right Product
Chris sheds light on the process his company went through in leveraging AI, sharing how the initial excitement surrounding GenAI wasn’t the right fit for their needs.
He points out the importance of focusing on the product first, rather than jumping on the latest technology trend. “We kind of figured out, well, which AI works the best for this product, and potentially down the road, some of the newer models that are kind of in the GenAI bucket could leapfrog what we sell now, but if you tried to do it now with GenAI, it really wouldn’t work that well.”
Chris’ company, which uses proprietary cameras to detect manufacturing defects, illustrates how combining AI with specific hardware can produce practical results.
Their embedded GPU cameras allow non-experts to leverage AI without needing extensive knowledge, simplifying the defect detection process in manufacturing. “They don’t need to understand AI really. They just need to kind of follow these prompts… it’s in the form that an engineer works in a factory wants.”
This focus on simplicity and usability in a non-AI-specialist context underlines the importance of choosing the right technology for a specific use case, rather than being swept up by the allure of new developments like GenAI.
Natural Inputs and Multimodal AI
Jae continues the discussion by bringing up the growing interest in multimodal AI, particularly when natural inputs are involved.
In physical contexts, such as driving or controlling machinery, traditional input methods like typing might not be the most practical.
Jae notes the need for AI to interpret more intuitive inputs: “You need to use your eyes, mouth, whatever, to input your intent, and you want the vehicle to understand you.”
The challenge of combining the physical and digital worlds is significant. Jae highlights that while GenAI excels in digital tasks, it’s not as effective in scenarios where physical inputs are involved.
This raises a critical question of when we want deterministic answers (clear, fixed results) versus probabilistic ones (answers based on likelihood).
In dynamic environments like driving, AI needs to balance safety with personalization, understanding when to take a creative, flexible approach and when to be rigid for safety reasons.
The Distinction Between GenAI and Other AI Applications
Praful wraps up the discussion by illustrating how GenAI differs from other AI models. In his own product, both GenAI and other forms of AI are utilized.
However, he emphasizes that GenAI’s tendency to hallucinate—generate overly confident but incorrect information—limits its usefulness in specific, data-driven applications. “For GenAI, I can just put a prompt saying, you know, write me a spec for sign up. And ChatGPT will hallucinate three pages of sign up… we don’t like that.”
By feeding relevant data into an AI system specifically designed for a workspace, the results are more accurate and tailored to the user’s needs.
This distinction underscores the importance of precision and context in AI development, reminding us that while GenAI has tremendous potential, it must be carefully managed and integrated with other AI forms for optimal results.
The Role of AI in Product and Investment Decisions: Insights from Chisel AI and Correlation Ventures
Praful highlighted the growing role of artificial intelligence (AI) in both product management and investment decisions.
The conversation focused on how AI, when leveraged effectively, can offer significant value—both in terms of improving user experiences and optimizing investment strategies.
This dialogue also brought valuable insights from Trevor (Audience), a partner at Correlation Ventures, which uses AI for venture decision-making. Let’s dive into some key takeaways from their conversation.
Chisel AI’s Predictive Assistant
One of the standout features of Chisel AI, as described by Praful, is their AI Assistant, designed to alert users to critical aspects of product development that require immediate attention.
Praful explained how this feature is particularly useful in detecting dependencies between features that could lead to potential problems if not addressed early. “It’s not like we’re just passing something to GenAI or some LLM,” he pointed out.
Instead, the model relies heavily on data to identify these issues. The challenge, however, is in training the AI model effectively.
Unlike general-purpose AI tools, the Chisel AI Assistant needs precise and accurate data to function at a high level.
“Without data, you’re not really going to get high quality,” Praful emphasized, drawing attention to a common misconception that AI can function well without robust data inputs.
The reality is that predictive AI tools need a strong foundation of relevant data to be effective, especially for tasks like anomaly detection.
The Power of Data in Venture Capital Decisions
The conversation then shifted to Trevor, who offered insights into how Correlation Ventures uses AI in venture decision-making. Their goal was to remove emotional bias from investment decisions while making the process faster.
AI is not intended to replace human-led due diligence but rather to supplement it, particularly for follow-up investments. Trevor shared that they use AI to streamline secondary investment decisions, reducing redundancy and the burden of repeated due diligence.
The AI-driven model they built focuses on three factors: the company, the investment round, and the investors involved. This model allows for more objective decision-making while keeping disruptive elements, such as unnecessary board members, out of the equation. As Trevor explained, “For check, new check, 2 through N perhaps you could use someone like us. So that’s what we’ve done. We’ve built an AI decisioning model.”
Building Proprietary Data Sets for Venture Outcomes
Trevor also elaborated on the importance of proprietary data in building effective AI models. Correlation Ventures not only purchased commercially available data sets but also developed their own through partnerships with VCs and other industry players.
They even took steps to contact thousands of companies to ensure their data was representative of the venture industry.
The key to Correlation’s strategy lies in proprietary data collection. As Trevor noted, “Do you build better algorithms, or do you have proprietary data? We think the algorithm piece probably will be harder to defend. So one of the strategies we’ve done is build the most complete dataset of venture outcomes data and protect that.”
While it remains to be seen which approach will ultimately prevail, Trevor expressed confidence that focusing on data completeness and representativeness offers a defensible strategy.
Ensuring Accuracy and Safety in AI-Driven Systems: Lessons from the Field
In critical industries like manufacturing and automotive, the need for precision in AI-driven systems is paramount.
Whether it’s about a self-driving car ensuring passengers’ safety or detecting defects in a production line, the consequences of inaccuracies can be severe.
During a discussion with experts from the field, several insights emerged on how they approach the challenges of AI implementation in high-stakes environments, where precision and transparency are non-negotiable.
Balancing Autonomy with Human Oversight
In the automotive industry, AI is used to create a safer, more autonomous driving experience, but human oversight remains crucial.
Jae shared his team’s experience working on an L2 autonomy system, which allows drivers to go hands-free but requires their attention on the road. The challenge lies in keeping users engaged without making them nervous about the technology. He noted:
“We use the user’s data to help prototype and improve the experience… but people get nervous. They get loud. So we look for ways to make it more ‘sticky’ and keep them in Blue Cruise longer.”
This careful balance between automation and user trust is achieved through continuous data analysis and design iteration, leading to features like auto lane-changing to address specific driving concerns.
Prioritizing Precision over Generalization
Chris emphasized the importance of high accuracy in AI models, particularly in use cases where the stakes are life-altering.
Unlike consumer-facing AI products like ChatGPT, which prioritize ease of use and general applicability, industrial AI systems need to meet stringent performance benchmarks. Chris explained the company’s approach to dealing with customers’ expectations:
“We haven’t shifted to GenAI because it’s more about labeling data and using older generation models that yield the precise results needed to make the product valuable.”
The goal is not just innovation for its own sake but ensuring the AI can deliver consistently reliable results, even if it means sticking with older, proven technologies over newer, more experimental approaches.
Transparency and Stakeholder Alignment
Mark shared his experience from GoodRx, where they implemented dynamic pricing using AI in a highly sensitive ecosystem involving retailers and patients. Transparency and clear communication were critical in gaining stakeholders’ trust. Mark highlighted three key components of their approach:
- Aligning everyone, from retailer partners to the executive team, on expectations.
- Defining clear tolerance ranges for errors—understanding that 100% accuracy is unrealistic, but setting acceptable thresholds like 99% for safety.
- Transparency in how data is being used and the potential penalties for incorrect decisions made by the AI.
“When we did all that, and then did the classic slow ramp, it actually worked out really well because people saw what the upside was.”
By taking a gradual, transparent approach, they ensured that all stakeholders understood the risks and rewards, leading to successful implementation even in a life-and-death context like drug pricing.
Continuous Testing and Validation
When it comes to manufacturing, rigorous testing of AI models is a standard practice. Chris described how manufacturers typically won’t trust an AI system until they’ve seen it accurately detect defects over multiple trials.
They focus on building trust by providing users with as much information as possible about how the model reaches its decisions.
“Manufacturing folks are rigorous with their testing… they might not put it onto their factory line until they’ve proven it can spot a hundred out of a hundred parts accurately.”
This meticulous approach ensures that AI systems can be trusted to operate under real-world conditions, where even a small error can have significant consequences.
Moreover, customers are encouraged to test the AI system’s performance before fully integrating it, allowing them to feel confident in its reliability.
The Evolving Role of Product Management in an AI-Driven World
As AI continues to reshape industries, product management is also evolving.
Automation: Shrinking the “Busy Work”
Product managers often juggle multiple responsibilities, from consolidating customer feedback to creating status reports and collaborating with different stakeholders.
This overwhelming “busy work” can take away from their core responsibility: strategic thinking. Praful, one of the speakers, emphasized that much of this administrative burden could and should be automated.
“A lot of this busy work could be automated. If you take all this busy work and shrink it, you make it easy for PMs to do that. Then they can actually do their day job, which is thinking about increasing customer adoption and driving revenue for the company,” Praful remarked.
He explained that the goal of tools like Chisel is not to reduce the number of PMs but to free them from mundane tasks.
Instead of being “middlemen” who ferry information between departments or compile reports, product managers can spend more time on what truly matters—crafting strategies that drive value and innovation.
AI and the Limits of Automation in Product Management
The conversation then shifted to whether AI could ever fully replace the role of a product manager. Could AI one day be smart enough to handle product development decisions or even generate new product ideas?
Jae posed an interesting question: “Do we think AI will learn enough to create product ideas that solve human problems better than humans can? And is that a world we want to be in?”
While the panel agreed that AI is making significant strides, they were unanimous in their belief that human intuition and creativity are still irreplaceable, especially in the context of product management. Praful was particularly firm on this point:
“I don’t think we are close to that state where you replace a product manager’s judgment or intuition. Creativity, thinking outside the box, and hustle—those are things AI can’t replicate.”
AI may handle data and automation effectively, but the strategic decisions that require a deep understanding of human needs and long-term vision are still best left to human PMs.
Addressing the Concern of Model Drift
Another technical aspect of AI in product management is model drift, a situation where AI models become less effective as time passes and the data changes. Chris, one of the panelists, touched on how they approach this issue in their organization:
“We give people exposure into the data and history of the judgments to give them confidence. But we don’t retrain based on results; if you’re going to retrain, you have to do it yourself.”
He explained that model drift hasn’t been a significant problem for them because their systems are designed to involve users in the process.
Their cameras can store up to 300,000 images, allowing users to sift through and cluster them, ensuring transparency and minimizing drift. However, they leave retraining decisions up to the users themselves.
The Future of Product Management: Striking a Balance
As AI becomes more sophisticated, it’s clear that the role of product managers is not going to disappear, but it will change.
Mark emphasized that while many of the more tedious tasks will be automated, the demand for PMs to bring their creativity and vision to the table will remain crucial:
“The role of product management should change. All the toil parts of the job will hopefully get better, but not every organization wants PMs to be aspirational leaders. In some cases, growth optimization might be well-suited for automation.”
What’s clear from the discussion is that automation will play a significant role in reducing the manual labor associated with product management.
However, the essence of the role—envisioning the future, making critical judgment calls, and understanding customer needs—will continue to require the human touch.
Embracing AI While Staying Strategic
A major challenge that businesses face today is the productivity of their teams, particularly in product management. One speaker highlighted the issue:
“We hire them for thinking about what kind of problems we should be solving, while they’re spending 90 percent of their time solving problems they probably shouldn’t be solving to begin with.”
This sentiment reflects a broader frustration in the field—PMs often get bogged down by tasks that could be automated, leaving little room for the strategic work that drives innovation.
AI presents a solution here by handling redundant tasks and freeing up time for more high-level decision-making.
As one business leader pointed out, the ultimate goal is to improve productivity and reduce time to market.
The Challenges of AI Adoption in Product Development
While AI holds promise, integrating it into product management workflows isn’t without challenges.
Chris discussed the current limitations of using AI for accuracy in product development:
“To get the GenAI model to be as accurate as the thing we have now is a lot of effort… We have a small team, and we decided not to put our energy into doing that just to make it a little easier to use.”
This reflects a cautious approach to AI adoption, where businesses recognize its potential but are not yet ready to invest significant resources into making AI a primary tool for complex decision-making.
The Impact on Product Teams: More Strategic, Less Routine
Looking ahead, the number of people needed to perform specific functions will likely decrease as AI becomes more integrated into workflows.
However, rather than replacing PMs, AI is expected to augment their abilities, allowing teams to be more strategic. As Mark noted:
“The changes are happening so fast… It allows for invention and scale at a pace we’ve never seen before.”
For product leaders, the challenge will be to remain open to these rapid changes while using AI as a tool to explore new possibilities.
The key will be balancing automation with the human intuition that remains crucial for product strategy and creativity.
The Human Touch in Design and Product Management: AI vs. Intuition
While AI offers efficiency and automation, there’s a growing debate about whether it can replicate the human intuition and creativity necessary for more complex tasks.
Jae, Chris, and Praful explored this dynamic, shedding light on the boundaries between AI and human judgment.
Jae provocatively pointed out the trade-off we face with technology’s acceleration. He referenced how the pandemic taught us the possibilities of remote work, reducing human interaction to “16×9 boxes” on a screen.
Yet, despite these technological advancements, Jae emphasized the need for slowing down, stating, “Humans are about slowing things down. That’s when humanity shows up.”
He highlighted that creativity thrives in the moments where there’s no clear formula, embracing the unpredictability and discomfort of a blank canvas.
Jae’s perspective as a designer goes deeper, drawing attention to how current tools prioritize efficiency at the cost of human spontaneity.
“We’re trying to microwave our way through everything,” he explained, pointing out that AI may offer speed but lacks the nuance that defines human innovation. For him, creativity isn’t about automating outcomes but reveling in the unknown:
“Every good company I went to where you were trying to crack this hard problem, there’s no formula. You have to go through the dark alleyway.”
Praful expanded on this idea in the context of product management, highlighting the limitations of AI in decision-making.
While AI can automate straightforward tasks, such as generating user stories or reports, he argued that it cannot yet replace the synthesis required in crafting a product roadmap.
“You have 100 user stories in front of you,” he said. “Somebody needs to synthesize this and have a point of view that out of these 100, only these six are important.”
For Praful, this synthesis—the ability to judge priorities based on the company’s current position and market context—is something that AI is far from mastering.
While AI may evolve to handle certain tasks, both Jae and Praful underscore that the human element—intuition, creativity, and judgment—remains irreplaceable.
Ethical Considerations and Risks of AI
Mark emphasized his worries about the potential for abuse and the unforeseen consequences of AI technology.
“I fear is just the abuse cases that are inevitably coming,” he noted, highlighting concerns about issues like deepfakes and misinformation.
He pointed out that while we haven’t fully witnessed the dark paths AI could take, the early signs suggest that significant challenges lie ahead.
“It’ll be interesting to see how the effect is on society in terms of how to react and how to trust or distrust,” he added, suggesting that public perception could have far-reaching impacts on the industry.
Chris contributed to the conversation by addressing the disparity between AI’s hype and its practical application within companies.
He remarked, “There’s so much hype about AI… but then when you’re pragmatically trying to figure something out, it’s not as great as it sounds.” This disconnect between expectations and reality can create obstacles for organizations trying to integrate AI effectively.
Jae raised another concern, stating that while technology can offer convenience, it can also foster a sense of laziness.
He noted the impact on younger generations, suggesting that as they grow up surrounded by technology, they might become less productive. “It’s not clear to me how that’s good,” he remarked, underscoring the importance of being mindful of our reliance on AI.
As these experts illustrate, while AI holds great promise, it also poses significant risks that society must navigate carefully.
Conclusion: The Future of AI and Human Creativity
As we delve deeper into the world of AI, it’s clear that while the technology holds tremendous promise, there are valid concerns that warrant our attention.
One of the most pressing issues is the potential for AI to foster complacency. For younger generations growing up with AI, social media, and smart devices, there’s a risk of becoming overly reliant on these tools, potentially leading to a reduction in productivity and critical thinking.
Another significant concern is the quality of AI outputs, which is heavily dependent on the data used to train these systems.
Poor-quality or biased data can result in misleading or inaccurate outputs, which might be mistakenly accepted as truth simply because they come from an AI.
This “garbage in, garbage out” phenomenon can have serious repercussions, especially in sensitive areas like public health or security.
Additionally, the explainability of AI decisions remains a challenge. Even when AI provides accurate results, understanding how it arrived at those decisions is crucial.
Without transparency in the decision-making process, there’s a risk of overestimating the reliability of AI, which could lead to costly mistakes.
Finally, while AI can be a powerful tool, it’s not without limitations. Current AI models are often transactional and lack the fluidity needed for creative processes.
The future of AI could indeed be bright if we manage to address these issues, particularly by enhancing the technology’s ability to support creativity and providing greater transparency.
As we navigate this rapidly evolving landscape, it’s essential to balance excitement with caution, ensuring that AI serves as a beneficial complement rather than a crutch in our lives and industries.
Check Out Full Event Here!