Learnings from Building AI Products
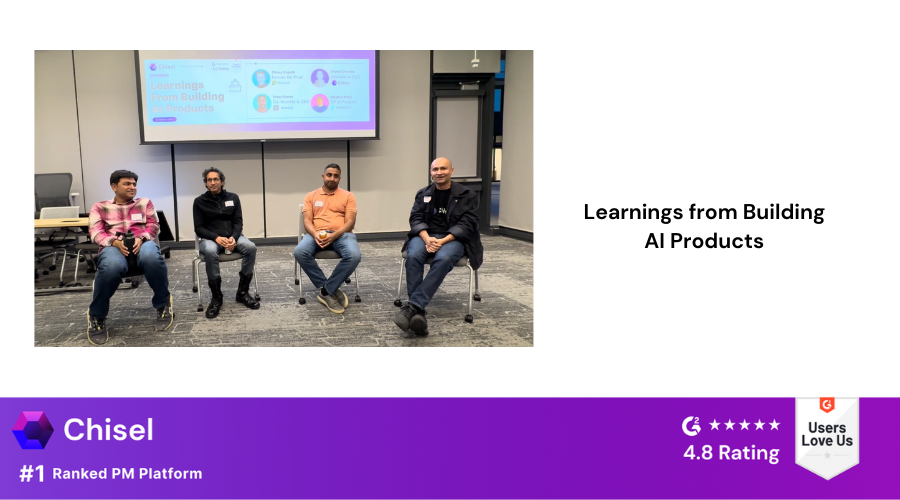
Welcome to an insightful discussion on Learnings from Building AI Products! If you’re a senior product leader looking to explore the world of AI through the lens of hands-on experience, you’re in the right place.
This panel brings together industry veterans who’ve not only built innovative AI-driven solutions but have also navigated the unique challenges that come with integrating artificial intelligence into product design.
Hosted by Praful Chavda, the founder and CEO of Chisel, the event offers a space for experienced product leaders to connect, exchange ideas, and dive deep into the AI landscape.
The session kicks off with introductions from panelists representing companie;.
1. Shiraz Cupala from Microsoft
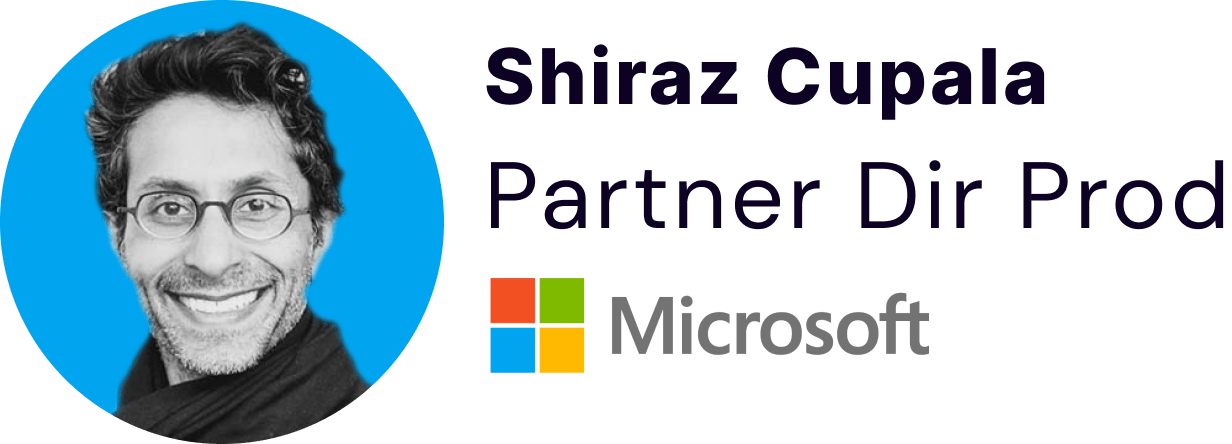
2. Meghal Patel from Truveta,
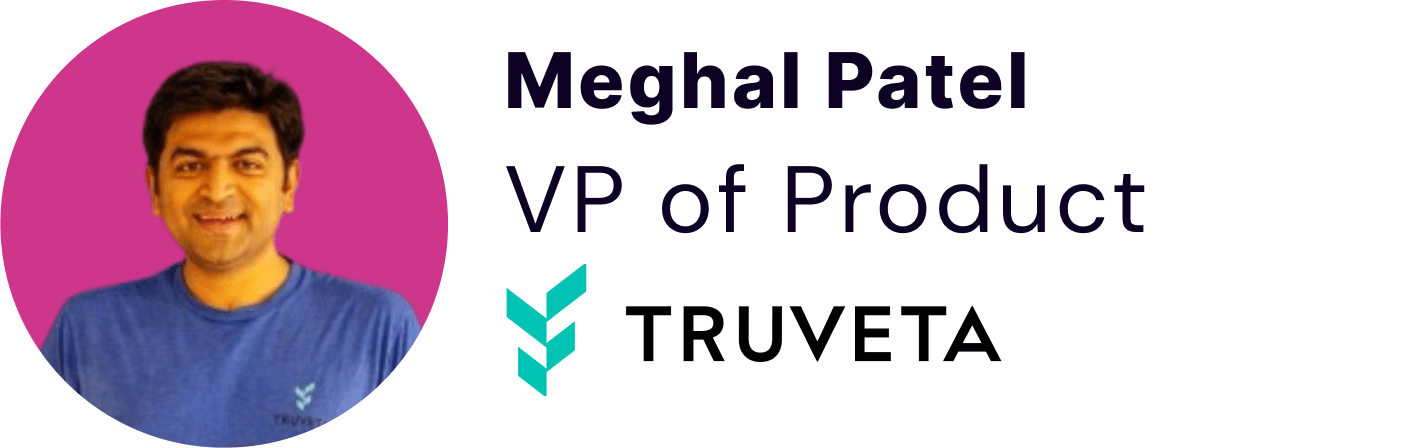
3. Prem Kumar from Humanly
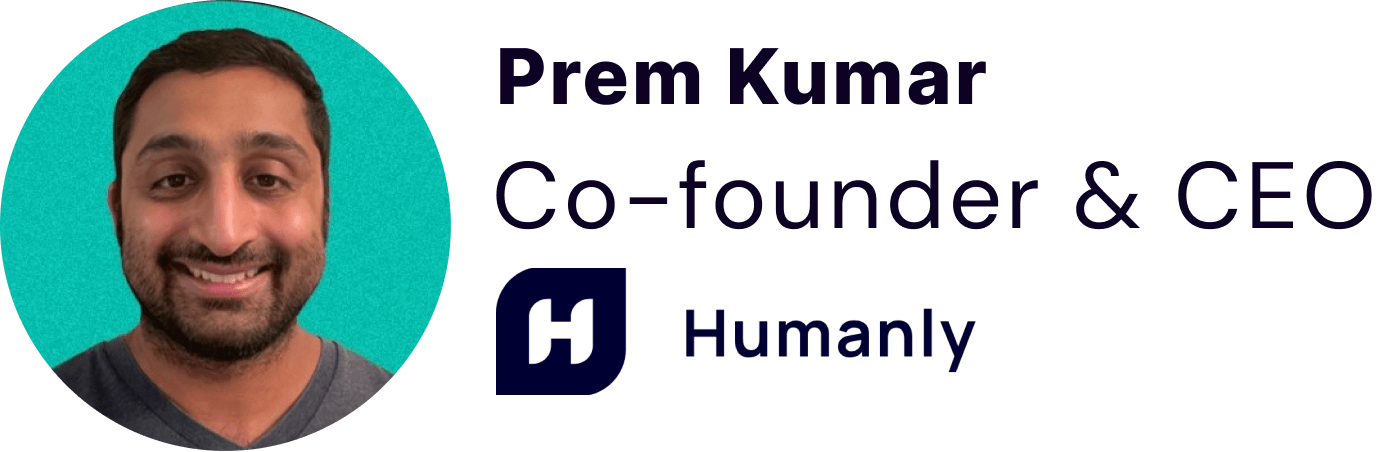
4. Praful Chavda the founder and CEO of Chisel
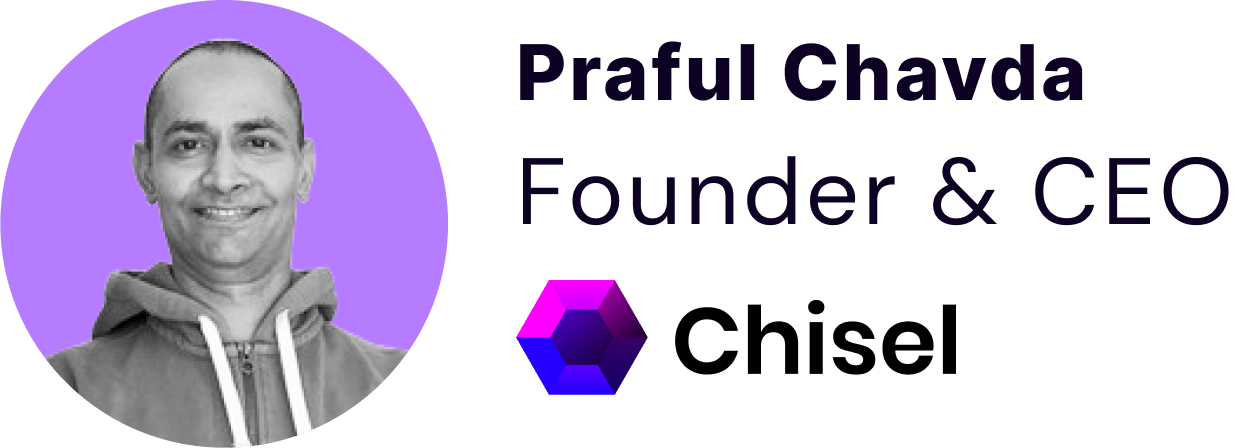
each bringing a wealth of knowledge from their journeys.
Whether you’re here to learn from the experts or contribute your own experiences, the conversation promises to be both informative and collaborative.
So grab a seat and get ready to delve into the future of AI product development.
Nature of AI Work
Meghal opens by explaining how they work with large healthcare systems to manage both structured and unstructured data.
In the past, organizing data required heavy coding, but with the rise of AI, they can now streamline these tasks through large language models.
A major focus at Truveta is on data normalization—standardizing information like names or medical conditions across different systems. Previously, much of this was done manually, but AI has allowed them to automate it efficiently.
He also discusses the challenge of extracting relevant information from doctors’ notes and other unstructured data, which AI now handles by making this data usable for research.
This automation allows healthcare professionals to pose questions in everyday language, even without technical expertise, which vastly improves their ability to analyze patient data.
Shiraz shifts the conversation by highlighting how AI has been an integral part of their work for years, although it’s evolved significantly.
Early uses included basic tasks like transcribing speech or changing virtual meeting backgrounds. However, with the introduction of large language models, AI has advanced to handle more complex tasks, such as better understanding human speech and behavior in meetings.
He explains that their focus now is using AI, through tools like Microsoft’s Copilot, to tackle long-standing problems more efficiently. The aim is to make these solutions more accessible, reducing the time and effort needed for users to achieve their goals.
Prem talks about AI in the hiring space, where it has the potential to revolutionize recruitment. Traditional recruiting methods often overlook most applicants, offering limited feedback.
Humanly is using AI to change that by providing conversational tools that allow candidates to interact with employers early on in the process. This approach gives every applicant a chance to engage, enhancing the candidate experience.
Furthermore, AI helps with matching the right candidates to the right jobs, enabling recruiters to focus on those who are the best fit, rather than spending time sorting through countless resumes.
Praful rounds out the discussion by detailing how they use AI in three key areas: document creation, predictive tools, and feedback classification. Whether it’s generating status reports or predicting project delays, AI is helping to automate essential tasks.
He also touches on how AI can sift through thousands of customer feedback entries, categorizing them so product managers can focus on the most relevant insights.
AI is not just a tool for innovation, but also a practical solution for making everyday processes faster, smarter, and more efficient across a range of industries.
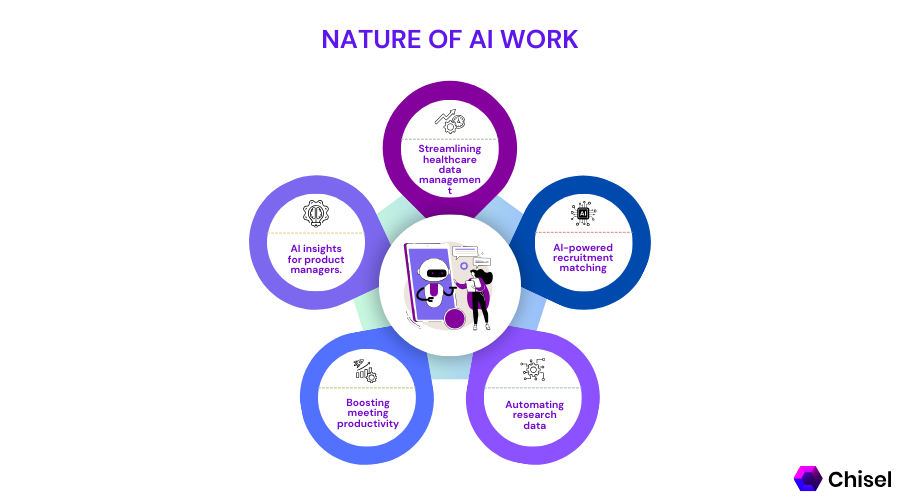
Building AI Products vs. Traditional Products
When it comes to building AI-powered products compared to traditional ones, a lot has changed in the product development lifecycle.
In the past, product teams would follow a linear, step-by-step process — from understanding customer needs, to building interfaces that guided users to a predictable outcome.
It was a more programmatic approach, where the focus was on teaching users how to interact with the system.
However, with AI, the process has become much more fluid and flexible. AI allows for non-linear problem-solving, enabling systems to understand human language naturally.
Users no longer have to “learn” the interface or structure their input in a specific way. Now, they can simply ask a question or make a request in their own words, even if it’s imperfect, and the AI will understand and respond accordingly.
This shift requires a new way of thinking. Instead of spending most of the time designing interfaces, the focus now lies on ensuring the output is trustworthy, accurate, and helpful.
In areas like healthcare, where trust is critical, developers must consider how to display responses in a way that instills confidence in users.
So, while AI simplifies the interaction for users, it also means product teams must rethink how they design and build their products — shifting focus from structured interfaces to ensuring AI delivers meaningful and reliable outcomes.
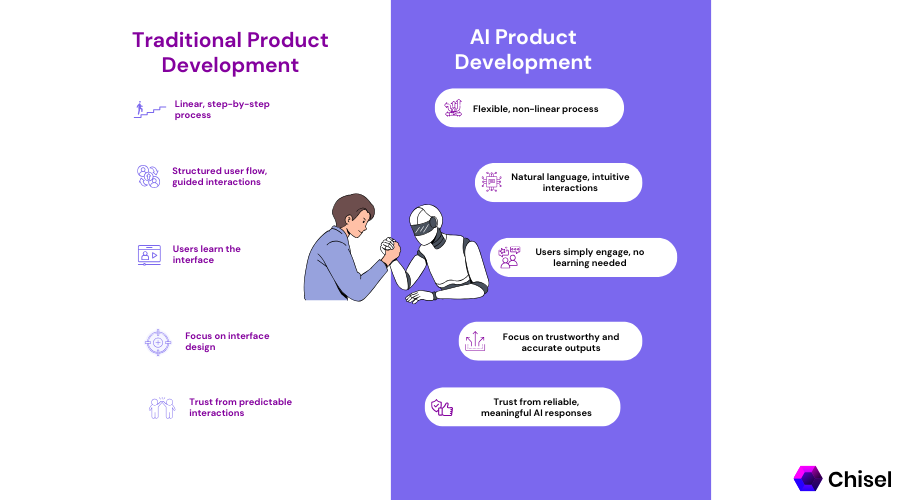
Challenges in AI Product Development
Startups in this changing environment face unique challenges and opportunities. A recent discussion highlighted the shifting landscape in product development, particularly for those venturing into the realm of AI.
One key takeaway is that the decisions about what not to build are increasingly critical. As Prem noted, with the commoditization of technologies like conversational AI, the real value lies in creating solutions that are not only functional but also tailored to specific contexts, such as understanding local laws or industry nuances.
The conversation also emphasized the importance of data. Unlike traditional products, AI-driven solutions hinge on robust datasets. Companies must ask themselves whether they have access to enough relevant data—be it proprietary or public—since the quality of AI outputs directly depends on the quality of the input data.
This shift necessitates a deeper understanding of data management and synthetic data generation, especially when real data is scarce.
Another significant challenge discussed was building trust with customers. While there’s a growing desire to use AI—83% of people express interest—trust remains a hurdle, with only 26% feeling confident in these technologies.
Startups, in particular, must navigate this trust deficit, as they are often seen as inexperienced players in a complex market.
Third-party audits and adherence to established frameworks, like Microsoft’s responsible AI guidelines, are steps companies are taking to reassure potential users.
The healthcare sector presents its own set of hurdles, where skepticism toward AI can run high.
Concerns about accuracy and reliability are paramount, especially in an industry where decisions can have life-altering consequences.
The unpredictability of AI responses adds to this challenge; healthcare professionals are understandably cautious, having experienced the disruptive impact of technology like Google in their practices.
Shiraz also pointed out, AI doesn’t behave like traditional software—it’s unpredictable. This lack of predictability complicates product management, as teams must find ways to define requirements and ensure outputs align with user expectations.
Establishing clear communication about the limitations and potential of AI is essential to fostering user confidence.
Ultimately, the path forward for startups in the AI space requires a multifaceted approach: understanding the intricate relationship between data and product quality, building robust trust with users, and preparing for the unpredictable nature of AI.
With these challenges in mind, companies can better navigate the complexities of creating AI solutions that genuinely solve customer problems and add value.
Impact of AI on Team Dynamics & Morale
One of the prominent challenges highlighted was the unpredictability of AI products. Meghal shared that his customer success team is feeling the strain as they navigate this uncertainty.
Unlike previous roles, where support could rely on predictable outcomes, AI products inherently come with uncertainties.
This has created tension between the customer success and product teams, as the former must manage user expectations when AI responses don’t align with what users anticipate.
Shiraz added that the skill sets required for teams have shifted significantly. The integration of data scientists into the team has become essential, as the reliance on data-driven insights is more pronounced than ever.
This shift means that product managers must adapt to an experimental mindset, constantly iterating and testing AI models to understand their capabilities and limitations.
The process of defining requirements has also become more fluid; teams must now adapt their strategies based on what the AI can realistically achieve.
However, Prem pointed out a silver lining in these changes: the need for collaboration with customers has increased. By involving customers in the design process, teams can develop solutions that better meet user needs.
This collaborative approach has transformed customer success roles from reactive to more prescriptive, allowing team members to provide insightful recommendations based on data analysis.
Despite the challenges, there’s a notable boost in team morale. Praful observed that working on AI products fosters enthusiasm among team members.
The opportunity to create innovative solutions has sparked greater initiative and creativity, leading to faster prototyping and experimentation.
In summary, while the journey of developing AI products is fraught with challenges, it also opens doors for collaboration, innovation, and heightened team engagement.
By embracing these changes, teams can not only navigate the complexities of AI but also harness its potential to drive meaningful advancements in their products.
Pricing and Value in AI Products
Once the discussion was open for questions, a key theme emerged regarding the unpredictability of AI outputs and its impact on unit economics.
An audience member pointed out that unlike traditional machine learning, which aimed for consistent results, today’s AI can produce unexpected outcomes.
This shift raises important questions about how companies gauge their costs and returns, especially when developing customer-facing experiences.
In the context of startups navigating these changes, it was noted that experimentation has become a vital part of the process. Companies are increasingly investing in research and development without immediate assurances of return on investment (ROI).
This was echoed by a Prem who mentioned reliance on powerful third-party services like Azure OpenAI, which can complicate gross margin calculations.
The infrastructure costs associated with AI are significantly different from traditional server and storage expenses, adding layers of complexity to financial forecasting.
Praful shared that Chisel is currently prioritizing customer adoption over unit economics. The focus is on delivering real value and ensuring customer satisfaction, rather than solely defining pricing strategies.
This sentiment resonates with many in the audience, as customers face similar challenges in establishing pricing for their own AI products.
Often, these pricing discussions become collaborative efforts, with customers sharing their insights and questions, making for a more engaging dialogue.
However, defining unit economics in this new landscape can be tricky. For certain AI applications, cost structures are more transparent; for instance, running models for specific tasks often has predictable costs.
Yet, the unpredictability of user engagement—whether a customer asks one question or fifty—adds an element of uncertainty.
This uncertainty complicates usage patterns and can lead to substantial costs, highlighting the need for continuous learning and adaptation within teams.
As companies grapple with these challenges, there is a noticeable shift in customer engagement strategies. Inviting customers into the design process has become increasingly valuable, helping teams move from a reactive stance to a more prescriptive role.
This collaborative approach enables a better understanding of customer needs and expectations, fostering solutions that are more tailored and effective.
One of the most compelling aspects of the conversation was the emphasis on delivering value rather than just focusing on cost efficiency.
Businesses must find innovative ways to add meaningful benefits for their customers, especially in an environment where AI can change rapidly.
The importance of understanding the customer’s problem—regardless of whether it’s tied directly to AI—remains central. The ultimate goal is to enhance customer experience, making the financial aspects secondary to the value being provided.
As the industry continues to evolve, it’s clear that balancing innovation with practical economic realities will be essential.
The conversation around unit economics and AI is just beginning, and it will be fascinating to see how companies adapt to these changes.
By focusing on collaboration, customer-centric design, and meaningful value delivery, businesses can position themselves for success in this dynamic landscape.
Language and Industry-Specific Challenges
Another participant raised an interesting question about the challenges of AI communication across different industries, particularly in construction.
They highlighted the importance of language that resonates with users and how crucial it is for AI tools to speak in a way that feels authentic and relatable to the audience.
Prem responded thoughtfully, emphasizing the varying communication needs across industries. He noted that, at their current stage, their AI chatbot can’t master every role or industry perfectly.
Sometimes, even within the same sector, the tone required for a senior position may differ significantly from that for a more junior role. It’s all about understanding the context and the audience.
He shared that while many users love the conversational style of tools like ChatGPT, research shows that around 90% of interactions with their chatbot yield better results when using rule-based responses.
For example, in construction, workers often prefer straightforward options—like pressing a button—rather than typing lengthy queries. This means knowing when to switch between natural language processing and more direct communication is key.
To ensure the AI provides relevant responses, Prem’s team leverages extensive data from various industries.
They often collaborate with customers to curate answers that align with their brand voice and values. If a query is more complex or doesn’t fit predefined answers, they might even consult a larger language model, depending on the quality needs of the situation.
AI Models and Customer Expectations
The speakers delved into how important it is to maintain a feedback loop when developing these technologies.
Prem emphasized that gathering user reactions—like whether they liked the answers they received—helps refine the AI’s responses.
Collaborating with customers from the very start is crucial; after the first month, they conduct reviews to ensure the AI aligns with user expectations.
Another interesting point raised was about running different AI models in parallel. Prem confirmed that they use a mix of homegrown solutions and Azure’s OpenAI. This flexibility allows them to cater to various customer needs based on the tier of service they require.
Next, Meghal shared insights about building their own large language model (LLM) from scratch, combining OpenAI’s tech with unique industry-specific data.
However, they also found value in using Retrieval-Augmented Generation (RAG), which can efficiently pull relevant information without needing to reinvent the wheel.
The discussion then shifted to the idea of using smaller, specialized models working in harmony, rather than relying on a single monolithic model. This approach allows for easier updates and improvements without the hassle of retraining a larger model.
Praful added to the conversation by explaining their decision to create a federated model. This gives customers the choice between various models, like Azure AI, based on their privacy preferences. It’s all about ensuring they don’t lose a deal due to model limitations.
Shiraz also weighed in with a practical example from his experience developing a co-pilot for meetings. Early on, the technology had a limited context window, which made processing conversations cumbersome.
Rather than forcing a solution that required heavy engineering efforts, they decided to let the technology evolve naturally. Soon enough, the capabilities improved, allowing them to handle longer meetings seamlessly.
The takeaway from this engaging exchange? It’s essential to adapt to the rapid advancements in AI technology while being mindful of user experience and operational efficiency.
Balancing immediate needs with future potential is the name of the game as these innovators strive to create products that resonate with users and meet their evolving needs.
Setting Context and Quality in AI Applications
Another lively discussion recently unfolded around the interplay between probabilistic and deterministic outputs in AI tools.
As more organizations embrace AI technology, understanding these concepts becomes crucial for both developers and users.
Let’s break down the insights shared during this conversation and explore how they impact our approach to product development.
Understanding the Concepts
At its core, the difference between probabilistic and deterministic outputs hinges on predictability. Deterministic models provide consistent results based on specific inputs—think of them as a straight road with a clear destination.
In contrast, probabilistic models introduce variability and uncertainty, offering multiple possible outcomes rather than a single answer. This can be especially challenging in product management, where stakeholders often expect precision.
One audience member expressed concern about how to effectively reduce the unpredictability associated with probabilistic outputs.
Their question sparked a fascinating dialogue about customer engagement and education. How do we ensure users are comfortable navigating this spectrum of outputs?
Empowering Users with Control
Praful emphasized a hands-on approach. He described how Chisel allows users to have a say in the classification of feedback. For example, when processing a batch of 400 feedback tickets, the system suggests tags based on its analysis.
However, recognizing that not all suggested tags will resonate with the user’s specific business context, the product offers the ability to modify these tags before finalizing the labeling process. This human-in-the-loop approach enhances accuracy and empowers product managers to make informed decisions based on their expertise.
Similarly, Shiraz noted the importance of striking a balance between creativity and precision in AI outputs.
While precise answers may feel more reliable, they can sometimes stifle the creative insights that come from human intuition.
This delicate balance requires careful consideration of what is appropriate for the customer, who may have varying preferences for rigidity versus creativity in AI-driven solutions.
The Evolving Customer Expectations
As customers become more familiar with AI capabilities, their expectations are shifting. Some customers prefer more structured outputs, while others crave flexibility and creativity.
This evolving landscape presents both challenges and opportunities for product managers as they navigate customer needs and desires.
One audience member poignantly described the struggle of determining when a product is “good enough” to ship.
The answer, according to Shiraz, lies in customer feedback. Engaging users in a back-and-forth dialogue allows teams to refine their offerings based on real-world experiences.
When customers express a strong desire for a product—almost to the point of needing it immediately—that’s when you know you’re ready to go.
Context is Key
Another important takeaway from the discussion was the significance of context in shaping product outputs.
Praful shared how their approach integrates context by allowing product managers to include relevant customer requirements and user stories when generating outputs like Product Requirement Documents (PRDs).
By embedding context into the AI’s processes, the outputs become much more aligned with the user’s specific needs.
In a similar vein, an audience member raised a common challenge in knowledge management: ensuring that teams reference the latest and most relevant documents.
Praful responded by highlighting how their system encourages product managers to actively select the documents and requirements they want to include.
This tailored approach helps avoid confusion and ensures that everyone is working from the most up-to-date information.
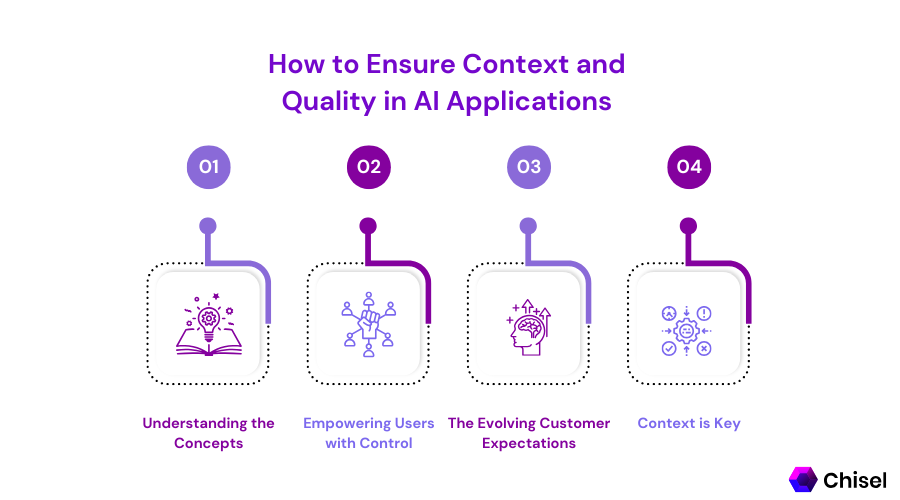
Conclusion – Customer Acceptance of AI Imperfections
As the landscape of product management continues to evolve, the expectations around AI-driven tools are shifting significantly.
Users are increasingly becoming accustomed to the idea that these tools may not always deliver perfect results but can provide valuable starting points. This acceptance marks a notable change in how teams approach product development and validation.
Gone are the days when every output was scrutinized to the finest detail. Now, there’s a growing understanding that AI can offer 80 to 90 percent of the necessary information, but human input is essential to refine and finalize the product.
This collaborative approach fosters innovation, allowing teams to leverage AI’s capabilities while still relying on their expertise to ensure accuracy and relevance.
Moreover, the acceptance of a certain level of error in AI outputs reflects a broader cultural shift towards agility and adaptability. As users interact with tools like ChatGPT and Microsoft Copilot, they learn to navigate the balance between automation and oversight.
This evolving mindset empowers product managers to experiment more freely, knowing that iterative improvement is part of the process.
Ultimately, the key takeaway is that the integration of AI into product management is not about achieving perfection from the outset but about enhancing human capabilities and fostering a more dynamic, collaborative environment.
By embracing this philosophy, teams can unlock new opportunities for creativity and efficiency, driving meaningful advancements in their products and services.
Here’s the Full Event Video:
Interested In More Of Our Exclusive Product Talks Events?