Data-Driven Product Management: Advanced Strategies for Analyzing and Leveraging Insights
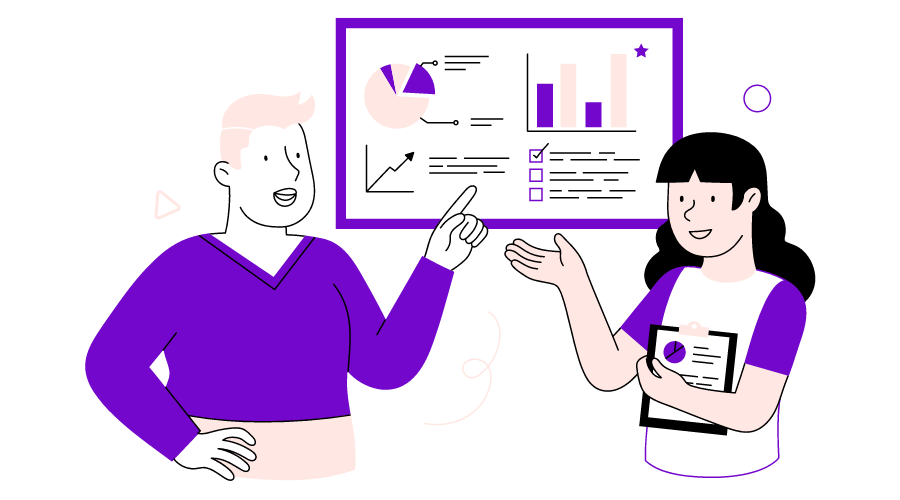
This resource contains,
- Advanced Data Analysis Techniques for Product Managers
- Harnessing Big Data for Product Innovation
- Leveraging Data-Driven Insights Across the Product Lifecycle
- Cultivating a Data-Driven Culture in Product Teams
- Case Studies: Success Stories in Data-Driven Product Management
- Future Trends and Innovations in Data-Driven Product Management
- Conclusion
When British data scientist Clive Humby declared “Data is the new oil” back in 2006, he was making two points: data is exceedingly valuable, but it also needs to be refined in order to be useful.
Almost two decades later, Humby’s analogy still resonates. In order to be successful, product managers and companies not only need to collect data, they also need to refine that data into insights, and then leverage those insights to make informed decisions that drive growth. And importantly, data collection and analysis aren’t just one-off activities. A truly data-driven approach to product management means that product managers need to operationalize their data by incorporating iterative testing cycles and continuous monitoring.
Data-driven product management strategies will make your team more agile and are essential for long-term success. Let’s examine some of the techniques product managers can use to collect, curate, and analyze data, and thereby make more impactful product decisions.
Advanced Data Analysis Techniques for Product Managers
With advanced data analysis techniques, product managers can distill vast quantities of customer data into actionable insights, and gain a deeper understanding of their customers’ needs and motivations.
When applied correctly, quantitative research can give product teams the ability to more accurately predict customer behavior, measure customer satisfaction at scale, and identify the product opportunities with the greatest potential return on investment. However, while quantitative techniques are useful, they must always be carefully qualified with research from other insights before making decisions. Combining the following techniques with traditional qualitative research will create a more well-rounded vision of the customer’s needs.
Predictive Analytics: Forecasting User Behavior and Product Performance
Predictive modeling finds patterns in large, complex data sets that can be used to identify risks and opportunities. By analyzing past usage data and known trends, predictive models can help product managers anticipate customer needs, forecast user behavior and product performance with a high degree of accuracy, and optimize products accordingly.
Applications for predictive analytics include forecasting new account growth, item adoption curves, or estimating the likelihood of users creating new content or activities on a service.
Some standard predictive analytics techniques include:
- Regression analysis forecasts continuous values like revenue or time on a website based on past behavior. Multiple regression looks at the impact of various variables.
- Decision trees Segment data into homogenous groups to predict categorical outcomes like churn, purchase, or failure. They help target interventions. Product management requires a lot of decision-making, and this can help tremendously with that.
- Neural networks – Inspired by brain processing, these sophisticated models can identify complex nonlinear patterns in massive data sets to make predictions. They are often used for image and speech recognition.
- Association rule learning – Finds relationships between frequently occurring variables to identify associations and correlations. Market trends and users evolve with time, and this learning can aid PMs in keeping in touch with user needs.
Once built and validated on historical data, predictive models can provide valuable insights to guide product decisions.
Sentiment Analysis: Turning Qualitative Emotions into Quantitative Data
Understanding customer preferences and satisfaction levels is crucial for product success, but asking customers to rate their satisfaction on a numerical scale can only get you so far. To really understand what customers are feeling, product managers need to examine how customers express themselves in their own words — in product reviews, social media posts, and freeform survey responses.
Sentiment analysis tools can analyze these kinds of qualitative artifacts in high volume and not only classify them by the most common sentiments expressed by customers, but also identify possible explanations for the most common sentiments.
Any product which relies heavily on customer reviews sits on a gold mine of data that is well suited for sentiment analysis. Product managers at travel booking sites, for example, use sentiment analysis on hotel reviews to identify specific attributes fueling positive or negative perceptions. They might find that free Wi-Fi and breakfast impact reviews more than room cleanliness, and in response, they can highlight these perks to boost bookings for hotel partners.
Cohort Analysis: Finding Opportunities through User Segmentation
Integrating cohort analysis into the product development cycle helps product managers continuously optimize their strategy. By reviewing how different cohorts engage with the product, upgrade, and churn, product managers can surface unforeseen issues or opportunities that one-off studies may miss.
Netflix extensively uses cohort analysis to see what makes some subscribers become more loyal and engaged than others. They analyze new subscribers by cohort to isolate different variables and study how user behaviors evolve. For example, they found that members who watched movies with more diversity in genres were less likely to cancel their subscriptions. This informed strategy shifts, like proactively recommending unexpected titles.
A/B Testing: Experimenting Iteratively for Continuous Learning
A/B testing provides a rigorous method for product optimization.
Research suggests qualitative feedback also offers insights incremental to quantitative testing. Combining both improves learning. Tests should run long enough to measure impacts beyond initial impressions. A 30-day window often separates transient effects from sustainable changes.
Randomization prevents selection bias but can be improved. Strategies like multi-armed bandits introduce variations sequentially based on interim results. This discounts unnecessary experiments fast.
Testing minor changes, even to small components, may significantly improve metrics. Changes causing 1-5% impacts are most common, though some produce 10-20% effects. Such learning happens through many successive experiments rather than single extensive overhauls.
Gradually refining understanding of user needs through repeated questioning incentivizes evidence-based innovation over untested assumptions. When new findings consistently point toward specific directions, product evolution accelerates.
Harnessing Big Data for Product Innovation
As a product manager, one of your most essential jobs is understanding your users and the market landscape so that you can build the right products. In order to do this, you need to create a repeatable process for collecting, managing, and analyzing data. Additionally, you need to be able to iterate on your process and make continuous improvements.
PMs must make data-driven decisions by determining relevant indicators, monitoring usage trends, and setting priorities based on verifiable information rather than conjecture.
Big Data Sources for Product Insights: Leveraging User Data, Market Data, and Beyond
Before you can make data-driven decisions, you need to have a sufficient amount of data, and you need the right data. With countless new data sources emerging daily, knowing where to start can take time and effort.
The most obvious place to start is with user data from your website, app, and other digital touchpoints, but going deep is essential. According to a McKinsey study, companies that incorporate big data and analytics into their operations see 5 – 6 percent higher production rates than their rivals. Take a closer look at metrics beyond just purchases or sessions. Examine event monitoring, site searches, flow dropout points, or trial discontinuation points to identify problems that cry for fixes. Collaborate with your analytics team to get valuable information from terabytes of usage trends.
Data from the market and industry provides a comprehensive picture of your domain outside of your four walls. Mitigate blind spots by staying up-to-date on competition intelligence, industry surveys, and third-party research.
Thinking outside traditional sources like reviews and surveys also yields nuggets. Demographic data or geo-tagged social media reveal unmet needs in certain regions. By blending disparate datasets, you can connect the dots between variables like weather patterns and online behaviors. Seek feedback from other functions, too, to cross-pollinate findings.
Product teams can get a strategic advantage by utilizing the abundance of big data and learning to search different sources for solutions. Use analytical rigor and think creatively to identify user needs and inform your product strategy. Breakthroughs are powered by daily information, so keep your curiosity high and do not restrict your quest.
Real-Time Data Analysis: Facilitating Iterative Product Development and Agile Decision-Making
The product manager’s critical task is to make data-driven decisions that continually enhance their products based on customer needs and market feedback. Continually improving a product is easier when your data is as recent and accurate as possible — which means that real-time data analysis is especially well-suited for an iterative approach to product development.
When combined with agile techniques and continuous product development concepts, real-time analytics can help validate assumptions, reveal problems, and improve product roadmaps by basing decisions on actual user behavior, rather than conjecture. Here are some possible applications of real-time data analysis:
- Monitor bespoke metrics such as time spent on specific features or conversion rates. Instead of waiting for monthly reports, you can quickly identify areas that need improvement.
- Real-time A/B or multivariate testing can identify the best design, copy, or flow. Make decisions based on facts to optimize the experience.
- When launching new pages or features, use surveys to get qualitative feedback. Consider insights before making a significant investment.
- Examine usage trends and typical routes. You can identify potential friction points or areas for personalization using real-time event streaming.
- Find errors or flaws as soon as possible from error logs, not after damage has been done. Implement updates in more rapid release cycles.
By acquiring customer behavior and interaction insights in real time, you can make better-informed decisions and support an iterative product development process.
Data Privacy and Security Considerations for Product Managers
A product manager’s job is to build the best products, but product managers are also responsible for upholding user privacy and data security. Adopting a privacy-first strategy is no longer optional—it is necessary for corporate success, given expanding legislation like GDPR and customers’ increasing interest in privacy.
The 1990s saw the development of Privacy by Design (PbD), a tried-and-true strategy. PbD is a process for integrating privacy safeguards into every stage of a product’s lifespan, from data processing after use to original design. According to studies, when used properly, PbD can assist in reducing over 90% of privacy risks. (2009, Cavoukian). We shall examine each of the seven PbD pillars in detail.
Considerations for Product Design: 65–80% of a product’s privacy and security features are decided upon during the first design phase (Stanley, 2016). Key areas to focus on include:
- Completing an evaluation of the privacy implications
- Defaulting to minimal data gathering
- Ensuring appropriate safeguards for systems and data
- Taking into account user privacy settings
Products should set up visible and safe processes for sharing, using, storing, and erasing data at every stage. Topics to concentrate on:
- Data reduction and restrictions limiting access
- Agreements for safe third-party data sharing
- Regular evaluations of access and timelines for data deletion
- User Informed Consent and Privacy
According to usability research, just 7% of consumers read privacy agreements (McDonald & Cranor, 2008). Simplified, layered, and just-in-time notification approaches are what product managers should use. Essential elements consist of:
- Detailed, voluntary consent processes
- Simple to use controls and settings for privacy
- Options for transparent information access and deletion
- Ongoing Evaluation and Enhancement
Perpetual PbD compliance is ensured in part by effect assessments and privacy audits. Subjects for ongoing evaluations consist of:
- Updated policies and alerts about privacy
- New forms of data or linkages with third parties
- New developments in technology or modifications to regulations
Product teams must constantly commit to implementing a privacy-first strategy. In today’s regulatory climate, this strategy also contributes to developing user trust, which is essential for corporate success. Product managers can use the concepts and considerations in this booklet to successfully integrate privacy into their work.
Leveraging Data-Driven Insights Across the Product Lifecycle
A truly data-driven strategy for product development integrates data collection and analysis in every stage of the product development lifecycle.
Product Discovery and Ideation: Using Data to Identify Market Needs and Opportunities
Effectively identifying market needs and opportunities is critical to developing successful products. In the product discovery and ideation stages, product managers can use early-stage techniques for gathering user data like focus groups and MVP feedback. Results from these practices can help inform go/no-go decisions regarding further development — saving you from headaches further on in the process.
Product Development and Iteration: Incorporating User Feedback and Analytics Insights
A data-driven, iterative approach to product development helps product managers continually refine their offerings. When paired with relevant methodologies and prioritization techniques, incorporating ongoing user feedback and analytics results in a virtuous cycle of optimization. This equips product teams to satisfy customers through each progressive release better.
Product Launch and Go-to-Market Strategy: Optimizing Launch Plans Based on Data Insights
Launching a new product requires meticulous planning and execution: an effective go-to-market strategy encompassing all launch activities is essential to the product’s success. Data-driven optimization is critical to success. The right pre-launch insights and ongoing refinement based on real-world metrics help product managers iteratively improve launch plans and performance.
Post-Launch Analysis and Iteration: Continuous Improvement through Data-Driven Feedback Loops
Post-launch evaluation is a critical process for product managers that facilitates continuous improvement. By analyzing post-launch data and implementing well-designed feedback loops, products can be iterated to meet user needs better.
Evidence-based decision-making is made possible by a comprehensive post-launch process that uses iterative feedback loops to incorporate a variety of quantitative and qualitative data sources. This promotes ongoing education and places goods in the best possible position to satisfy changing consumer demands in a dynamic marketplace. Product managers can further improve evaluation techniques to promote continuous improvement with close supervision.
Cultivating a Data-Driven Culture in Product Teams
In today’s data-driven business environment, having product teams that can effectively analyze data and leverage insights is crucial for success.
Building Data Literacy: Training Product Teams to Interpret and Utilize Data Effectively
The capacity to gather, handle, evaluate, and present data in a critical, morally driven way is known as data literacy. It includes technical skills like data visualization and database querying and soft skills like pattern spotting, evidence-based decision-making, and critical thinking. Product teams can significantly increase their effectiveness by investing in data literacy training.
According to research from Gartner, just 27 percent of companies evaluate their staff as having high data literacy. Ineffective data literacy impedes management and product development in several ways. It results in inadequate data hygiene, lost opportunities for insights, inadequate effort prioritization, and incorrect product strategies.
Product teams that are well-versed in data literacy can benefit companies by:
- Improving outcomes: Data literacy, based on usage analytics, surveys, and other data, assists in concentrating resources on features and challenges that are most important to customers. As a result, product-market fit is enhanced.
- Aligning tactics with results: Data literacy enables teams to use experiments and A/B testing to correlate tactics and modifications to results. By doing this, wasteful attempts are avoided.
- Remaining adaptable and agile: Data literacy enables teams to promptly analyze vast, complicated data for problems and possibilities, keeping them sensitive to the market’s changing demands.
- Boosting accountability and openness: By regularly sharing KPIs and metrics, data literacy encourages evidence-based conversations across teams and with leadership.
Fostering Collaboration between Data Scientists, Analysts, and Product Managers
As data becomes increasingly important in shaping business decisions, product success depends on encouraging collaboration between product managers, data scientists, and analysts. However, studies reveal that many organizations find it difficult to dismantle the functional silos that exist between these roles.
Some of the benefits of cross-functional collaboration include:
- Better insights: Collaborative ideation facilitates the mapping of analytical results to particular product issues, improving clarity.
- Improved A/B testing: For iterative improvements, teams collaborate on creating hypotheses, designing experiments, and analyzing results.
- Setting priorities strategically: Cross-pollination of tactical and strategic viewpoints makes it easier to prioritize the 20 percent of efforts that yield 80 percent value.
- Shortened time to market: Parallel working shortens product development cycles by preventing hand-off delays.
- More innovation: At the nexus of technology, consumer needs, and company objectives, different perspectives give rise to fresh concepts.
Collaboration across data, analytics, and product management is essential to insights-led development. Strategic cross-functional models foster the common understanding and social ties necessary for utilizing various skills to maximize experiences, goods, and commercial results. Frequent talent exchanges enhance the collaborative culture even more.
Establishing Data-Driven KPIs and Metrics for Product Success
Product teams can improve decision-making at any point in the product lifecycle by monitoring the appropriate metrics and key performance indicators (KPIs). Evidence-based decision-making requires careful consideration of the customer, company, and product KPIs about the goals and stage of the product.
Product teams can increase the likelihood of a successful product by using qualitative and quantitative research methods to track leading and lagging indicators. This process allows for iterative improvement. Starting with the establishment of a data-driven approach sets the stage for ongoing innovation and growth.
Case Studies: Success Stories in Data-Driven Product Management
Product managers need to be able to use data to drive business growth and optimize product strategy. Let’s examine the following successful cases of product managers using data-driven techniques to improve their decision-making.
Case Study 1: Netflix’s Data-Powered Programming Decisions
One of the earliest big success stories in data-driven product management has been Netflix. Before their popular recommendation system, Netflix executives made strategic programming choices based on large-scale customer data analyses. For example, data showed that the British version of “House of Cards” was popular among a demographic that research indicated would also respond well to an American adaptation starring Kevin Spacey and David Fincher. On this evidence alone, without seeing a pilot, Netflix offered significant contracts for the now acclaimed series.
Netflix has a massive competitive advantage over traditional television networks because they are able to greenlight projects that algorithmic models indicate will perform well, rather than relying solely on creative instincts. This data-driven approach is estimated to have saved the company millions in unnecessary pilot production costs over the years.
Case Study 2: Uber’s Dynamic Pricing and Demand Forecasting
Another example of data-driven product management is Uber, which owes much of its success to creative applications of data. A core challenge for Uber and similar ride-hailing platforms is matching highly fluctuating rider demand with driver supply patterns across urban geographies. To address this, Uber developed “temperature maps” using algorithms that analyze historic request volume data to identify hotspots for rider pickups at different times. This guides drivers efficiently to high-demand locations instead of sitting idle elsewhere.
Additionally, Uber leverages machine learning models to adjust pricing based on real-time demand forecasts. During surge periods, algorithms raise rates just enough to incentivize more drivers to go online without losing too many riders. Over time, Uber refines these models using petabytes of operational data from over 100 million monthly active users globally. This data-powered optimization of prices, driver supply, and rider matching is a core reason for Uber’s growth despite challenges from the traditional taxi industry.
Case Study 3: Data-Driven Decision-Making at Anthropic
A more recent example of data-driven product management techniques comes from the AI company Anthropic. The Anthropic product team encountered complex technical challenges when developing their self-supervised Constitutional AI techniques. By leveraging their research data, comprehensively tagging metadata, and performing rigorous empirical evaluation of models, they identified failure modes to improve training procedures.
A/B testing across multiple research pipelines further uncovered the most sample-efficient curriculum components. Statistical analyses of how AI assistants responded to edge case prompts revealed subtle biases to mitigate. Bringing data science acumen internally equipped Anthropic’s product managers to iterate rapidly based on measurable metrics from petabyte-scale research datasets. This data-first methodology is argued to have significantly accelerated their progress in developing beneficial artificial general intelligence capabilities.
As seen in these case studies, thoroughly integrating data analysis principles into product management enables evidence-based decision-making at a massive scale. The ability to visualize customer behaviors and technical processes, identify patterns, and quantitatively measure impact equips data-driven product teams to solve problems faster and unlock new growth opportunities. While data science capabilities are now table stakes for product managers, those who build data insight into their day-to-day workflows can gain substantial competitive advantages for their organizations.
Future Trends and Innovations in Data-Driven Product Management
Technological advancements, changing markets, and evolving customer expectations drive constant changes in revolutionizing product management. Data is becoming an increasingly important factor in business decision-making as digital technologies continue to infiltrate economies and societies. How will new data sources and analytics shape the future of the product management industry?
Taking Advantage of Data Analytics’ Power
Studies show that data analytics plays a growing role in strategic product choices. According to recent polls, more than 90% of product managers routinely use analytics to optimize different work areas. Product teams can obtain unparalleled insights into user behavior, product performance, and market dynamics using machine learning and predictive modeling.
Returning to the example of the AI company Anthropic: they created personalized chatbot chats by analyzing usage patterns from over 500,000 clients. As a result, they more than doubled user retention rates. Other businesses, like Spotify, have included analytics at every stage of the development process, from conception to feature prioritization to post-launch monitoring. These data-driven strategies are becoming standard because they increase productivity and customer value.
Emphasize personalization and user experience
According to recent research, 70% of businesses intend to expand their spending on user experience (UX) design in 2023, indicating that UX design will be a top priority (Forbes, 2022). Businesses use data to understand people better and customize experiences in response to the growing desire for intuitive, seamless digital products.
For instance, over time, Netflix used machine learning on more than 100 million user profiles to improve the platform’s interface and content recommendations. Comparably, Amazon evaluates more than 2.5 petabytes of user data daily to autonomously customize the fulfillment processes and shopping experiences for more than 200 million customers. These data-driven, customer-focused initiatives are crucial differentiators in fiercely competitive marketplaces.
Emerging Technologies drive innovation
Machine learning and artificial intelligence have enormous potential for creating ground-breaking product capabilities. Businesses are investigating uses for digital therapies driven by biosensors and genetic data analysis, computer vision for driverless cars, and predictive maintenance in the industrial Internet of Things.
General Motors’ OnStar uses AI to track thousands of car sensors and identify irregularities, which helps anticipate and stop mechanical issues. DeepMind’s AlphaFold algorithm overcame the 50-year-old protein folding problem through self-supervised learning, creating new opportunities for revolutionary medical advancements. In the ensuing decades, such developments at the nexus of developing technologies and human demands will redefine the potential of products.
Product teams that want to stay ahead of the curve and manage constant disruption must adopt data-driven tactics and a research-oriented mindset. Achieving success in this dynamic profession now requires continuous learning and trend adaptation. Even though many issues with data privacy, ethics, and environmental sustainability still need to be resolved, product managers and innovators have a great deal of responsibility and excellent chances to improve people’s lives through technology in the future.
Conclusion
Product managers need a data-driven mindset and constantly approach their work to learn more from customer feedback. Long-term product success will depend on our ability to use analytical approaches to provide tangible data to back our beliefs, even if experience and intuition are always necessary. We implore everyone to investigate methods for obtaining further qualitative and quantitative user feedback and to form partnerships with data scientists who can help transform those raw inputs into insightful findings.
The widespread use of digital products and services will only increase the importance of data-driven decisions. Product management is evolving into a strategic discipline that balances rationality and creativity.