How to Conduct User Sentiment Analysis In 2024?
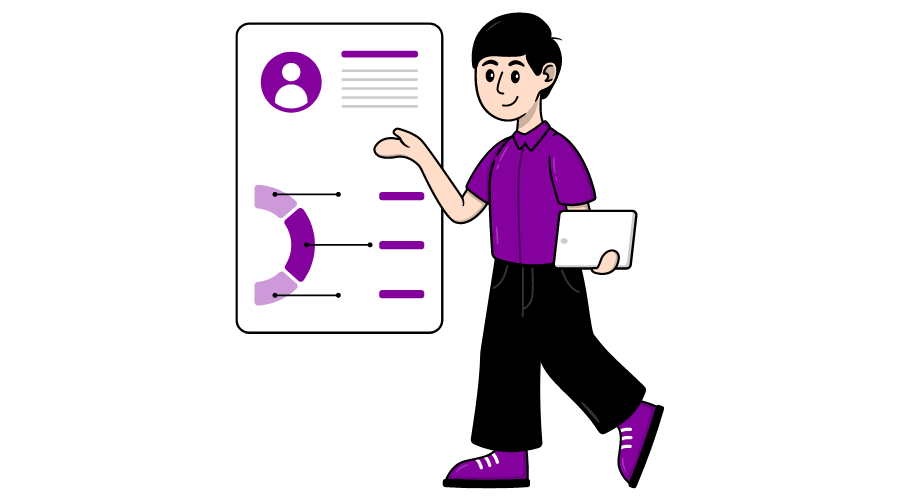
Are your customers happy or having problems you don’t know about? Understanding user sentiment is crucial for business success. However, making sense of vast amounts of unstructured user feedback can feel overwhelming.
In today’s digital world, where over 50% of consumers share their thoughts across myriad online channels, according to IBM, truly understanding what your users think and feel can seem nearly impossible. That’s why analyzing customer sentiment has become a must-have capability for forward-thinking brands.
User sentiment data holds a gold mine of insights into the customer experience. However, most companies are not effectively tapping into this rich source of information about what matters to users.
By 2024, those who can analyze sentiment at scale and take action will gain a competitive edge. This guide helps you join that leading group with a step-by-step process for unlocking the hidden feelings in your user data.
Learning how customers truly feel beneath the surface of their words is key to addressing pain points, retaining users, and growing loyalty. Let this article be your starting point for leveraging one of the most powerful yet underutilized techniques for business success.
Why Sentiment Matters?
Understanding your users’ perspectives is key to making informed business decisions and improving experiences. Sentiment analysis provides a window into customers’ thoughts and feelings through objective insights from open-ended feedback. Using machine learning and natural language processing techniques, brands can extract emotions from text to achieve important goals.
One of the main benefits is gaining clarity on satisfaction levels. Analyzing comments reveals the root causes driving certain ratings, whether confusion, frustration, or consideration of alternatives. It allows companies to prioritize targeted improvements with a big impact. For an upset mobile user, fixing an interface issue may retain them. At the same time, a discount could sway someone on the verge of switching platforms.
Sentiment analysis also furnishes the context behind user behaviors. Beyond just what actions people take on a site, understanding motivations and pain points provides a fuller picture. For instance, supplementing session recordings with feedback can uncover why some visitors abruptly leave certain pages. Identifying such friction points enables swift, optimal changes to reduce drop-offs.
Bringing the voice of the customer into strategic discussions helps align cross-functional teams. Disagreements over needs get replaced with a shared perspective grounded in qualitative consumer insights. This evidence-based approach to decision-making facilitates product-market fit and growth over-relying on assumptions.
Additionally, sentiment yields clues about emotional investment. Higher attachment indicates stronger loyalty, repeat purchasing, and willingness to recommend. Targeting these devoted customers supports marketing initiatives to boost engagement through discounts or membership perks.
Brands also leverage sentiment to monitor discussions and notice if messaging resonates as intended. Adjusting campaigns promptly maintains relevance. Automating data organization with sentiment tools expedites obtaining actionable conclusions from expansive inputs.
Ultimately, regularly analyzing sentiment furnishes an empathetic understanding of how experiences make people feel. This insight fosters products that improve lives, strengthening beneficial relationships between businesses and their supporters.
What Is User Sentiment Analysis?
User sentiment refers to customers’ positive, negative, or neutral emotions and opinions about a brand, product, or service. It provides insights into how customers feel rather than just what they do.
For example, a negative review saying “the interface is hard to navigate” suggests the user is unhappy with the product design. Or hesitant language in a sales call could indicate doubt. Positive sentiment would be signaling enjoyment, liking features, or praising the brand.
Companies can understand the root causes driving satisfaction levels by analyzing sentiment from these unstructured data sources. They gain a well-rounded view of how customers emotionally connect with the experience instead of just top-line metrics. This context helps businesses better target improvements and enhance the overall customer experience.
Understanding customer sentiment is critical to improving one’s product or service. While AI and NLP can recognize emotions in text, different analyses are needed to grasp customer perspectives fully. Let’s explore four common approaches.
The fine-grained model provides a nuanced view. It categorizes sentiments as negative, negative, neutral, positive, or positive. It gives detailed feedback, especially for reviews. Scales from 1 to 5 or 1 to 10 can also represent varying intensities, with lower numbers signaling stronger negativity and higher signaling positivity.
Aspect-based analysis delves deeper. Beyond overall polarity, it identifies specific topics that people discuss. Take an app review mentioning a “clunky” and “difficult to use” interface – these pinpoint issues warrant attention. Segmenting sentiments by aspect gives actionable insight into improvement areas.
Emotion detection considers feelings beyond polarity, like sadness, anger, or happiness. Lexicons listing emotive words can help, but machine learning better handles varying expressions.
Intent analysis provides another helpful lens. It gauges whether individuals intend to purchase or just browse. For interested customers, targeted ads can encourage sales. Don’t unnecessarily pursue on-buyers, saving resources. Understanding intent helps optimize marketing effectiveness and efficiency.
Imagine you run an online retail store. Your most recent sentiment analysis showed that customers were generally satisfied with their shopping experience and purchases. On the surface, this seems positive.
However, relying only on that single data point could lead your business into a false sense of complacency. Sentiment analysis is most useful when tracked over an extended period.
Say subsequent monthly analyses six months later reveal a slow decline in customer satisfaction scores. Even though the ratings are still positive on average, this downward trend is an early warning sign.
Now, prompt yourself to examine the data to identify potential causes. After examining sentiment ratings alongside specific customer comments, you notice increasing frustration with shipping speeds and order delivery times.
Armed with this insight from longitudinal sentiment tracking, you can make quick improvements like optimizing your fulfillment process and logistics. Customer satisfaction scores immediately bounce back up as a result.
Channels Where User Sentiment Can Be Found
Customers now have many avenues to express their opinions; sentiment analysis must account for these varied channels. Some examples:
- Customer surveys directly capture satisfaction levels, but people may hold back. Online reviews offer unfiltered feedback.
- Chatbots and help desks reveal frustrations in real time. Analyzing transcripts shows if specific issues repeatedly cause negative emotions.
- Sales call analysis can detect subtle hesitations a person may not outwardly express. It helps reps address concerns before losing a sale.
- Social media is rich with unprompted opinions. Tracking sentiment on platform mentions and posts provides an outside view of brand perception.
- Comment sections let users react openly to new products/prices and more, providing an early warning of potential issues.
Gaining insights from this wide range of sources paints a well-rounded picture of the customer experience journey. It ensures no negative signals from any individual channel are missing. Businesses need to integrate sentiment data holistically from all touchpoints to understand evolving customer needs and satisfaction over time fully. A multi-channel approach is essential today.
6 Steps to Conduct User Sentiment Analysis
- Collecting Data
- Organizing and Cleansing Data
- Examining Data
- Creating Visualizations and Sharing Insights
- Implementing Findings
- Testing Modifications and Confirming Insights
Step 1: Collecting Data
Collecting the right data is the first step in conducting accurate user sentiment analysis. Customer feedback gets scattered across many sources, like online reviews, social media posts, emails, and support tickets. The key is gathering all this unstructured data into one place for analysis.
Some ways to collect user sentiment data include:
- Surveys: Put targeted surveys on your website or app to get direct feedback from customers on specific topics.
- Online Reviews: Integrate review plugins on your website and monitor third-party review sites. Be sure to respond to reviews to build trust and gather more data over time.
- Social Media: Use APIs to pull posts about your company or product from platforms like Facebook, Twitter, Reddit, and more. Look for mentions, hashtags, and conversations related to your brand.
- Support Tickets: Analyze the text of support emails and in-app chat logs for tone and common pain points customers experience. Make notes of positive and negative sentiments expressed.
- Forum Discussions: Monitor industry forums and groups for unprompted discussions about your solution. Look for keyword alerts.
If you are a product management company, sources like sales demos, implementation feedback calls, product onboarding surveys, and success story interviews provide rich qualitative insights beyond reviews alone. Make sure to gather this internal data in addition to external commentary.
The goal is to cast a wide net to collect a robust dataset across all relevant sources. This varied user feedback forms the foundation for identifying patterns and trends through sentiment analysis tools.
You can create different audience panels in Chisel to survey and collect input from specific user segments. It allows analyzing sentiments across user types.
Step 2: Organizing and Cleansing Data
Before analyzing any data, it needs to be organized and standardized so artificial intelligence systems can properly interpret it. Text often contains emojis, slang, incorrect punctuation, and other elements that confuse algorithms. The data must be “normalized” or structured in a consistent format.
To enhance data cleanliness, one method involves eliminating stopwords, common language words like “and” and “but” that lack meaningful context. Adjustments to punctuation are also essential, and specialized text cleaning tools are available to scan content and implement these formatting changes automatically.
Achieving similar data preprocessing tasks is possible through Python code. This normalization process aids in improving AI’s understanding of the core sentiments you wish to convey.
For diverse data types, such as video, audio, or multiple languages, additional preprocessing might be necessary. Transcription, converting speech to text, facilitates analysis, while translation standardizes international content to a language supported by the tools.
Normalizing transcription data from phone calls is essential for a product management company to collect customer feedback. Conversation analysis software can record and transcribe support discussions. Text cleaning removes non-essential elements, prepping the verbatim comments for sentiment review. It reveals which customer service interactions went well and where frustrations occurred, helping the business continuously improve.
Awareness of differing dialects is also key, as some expressions may get misinterpreted without context. Choose sentiment analysis software capable of adapting to unique vernaculars used by various demographic groups within your user base. It leads to more accurate sentiment scoring across all customer conversations. Proper data preparation lays the foundation for insightful user sentiment insights.
Chisel employs AI/ML techniques to handle both structured and unstructured data, incorporating features such as text analysis, synthesizing feedback tickets, and more. This aids in normalizing diverse formats of qualitative data from various sources into a standardized structured format for comprehensive analysis.
Step 3: Examining Data
Once you’ve gathered all your user sentiment data through surveys, social media, reviews, and other channels, it’s time to make sense of it all.
The first step is to organize the raw data so it’s easy to digest and draw insights from. You’ll want to use text analysis software to automatically tag and categorize responses by common themes for qualitative data like open comments. It allows you to spot trends in what users are saying easily.
The software can calculate average sentiment scores for different parts of your product or service for quantitative data like rating scores. You can then segment the data by user type, location, purchase history, and more to see how sentiment may differ between groups. This level of analysis enables you to pinpoint exactly where improvements need to occur.
Many companies use specialized sentiment analysis platforms tailored to their industry to perform this data crunching. Providers like G2 and TrustRadius allow you to analyze reviews left on their marketplaces. After uploading your data, the tools first “train” themselves by having users manually categorize a sample to learn common language patterns.
They can then automatically assign tags to future submissions, flagging usability issues and technical problems or praising for support. Aggregating sentiment scores lets you gauge overall satisfaction levels over time. With filters, you can isolate sentiments from power users or large enterprise clients to meet their unique needs. With these actionable insights, product and marketing teams can make well-informed decisions to enhance the customer experience.
Chisel’s Idea Box could serve as a centralized repository to collect, organize, analyze and draw meaningful insights from user feedback data to help optimize the customer experience. The categorization and filtering tools help make sense of large amounts of qualitative and quantitative feedback.
Step 4: Creating Visualizations and Sharing Insights
Presenting findings through clear visuals like graphs and charts helps stakeholders understand the key takeaways at a glance.
Many sentiment analysis tools provide dashboards that consolidate all your meaningful metrics and feedback onto a single page.
Proper visualization is crucial to getting buy-in for recommended changes. Display the sentiments expressed and dig into the root causes driving those emotions. Identify which specific areas or features are problematic according to user feedback. Tools like session recordings can help uncover what exactly frustrated or confused users.
Don’t just share results internally – communicate insights to cross-functional teams, too. A marketing team analyzing a new campaign may find product-related comments to pass along. The Chisel + Slack integration streamlines this process, sending alerts to relevant channels.
Let us say a company is launching a new CRM tool. The sentiment analysis would reveal some aspects scoring higher than others. By filtering their dashboard, the team will see billing and support received lower satisfaction than features or usability. It will point them toward prioritizing fixes to those less favorable elements first. Presenting a breakdown of individual metric performance will empower data-driven decision-making.
Step 5: Implementing Findings
The culmination of the sentiment analysis process is taking action based on the insights uncovered. Improving the user experience should be the ultimate goal, so implementing identified changes is paramount.
Sentiment tools often classify exactly which aspects or features elicit negative reactions. This pinpoints root causes and opportunities for optimization.
To implement findings effectively:
- Prioritize changes aligned with business objectives and user needs
- Involve cross-functional teams in changes outside a single department
- Test modifications through additional sentiment analysis to ensure meeting goals
- Monitor metrics after launching updates to confirm improvements stick
Let us say you are a SaaS startup, and your analysis uncovers that the onboarding process on mobile devices is taking too much time. You decide to streamline the signup process by reducing fields and introducing one-click inputs, resulting in a notable boost in conversion rates.
To further enhance user experience, you integrate more seamlessly with identity providers, allowing broader access while maintaining security measures. Upon re-evaluation, it becomes evident that these tweaks have successfully achieved the intended improvement in user satisfaction.
Chisel offers an exclusive ‘Team Radar’ pillar for ensuring that the voices of all team members are heard and taken into account during prioritization and decision-making processes. It reveals the alignment or divergence of team members’ priorities.
Step 6: Testing Modifications and Confirming Insights
The final step in successful user sentiment analysis involves testing the changes and validating that those updates achieved the desired outcomes. Continuous testing and evaluation ensure the insights gathered translate to improved experiences and greater customer happiness.
Some methods for testing changes and validating learnings include A/B testing interface variants, surveying customers post-update, and analyzing metrics over time.
Taking these testing and validation steps as an ongoing part of the process helps optimize the returns on investments from sentiment data. It prevents wasting resources on improvements that didn’t enhance user satisfaction. The insights continuously provide feedback on future product planning and strategic decision-making.
Conclusion
As technology advances at lightning speed, companies need to pay close attention to user sentiment to keep up with changing needs and preferences. While conducting user sentiment analysis can provide valuable insights, it is not always easy or affordable for many businesses to implement robust sentiment analysis programs independently.
Thankfully, Chisel provides a user-friendly platform that makes sentiment analysis accessible and affordable.
Through the simple yet powerful web app, Chisel can help you effortlessly track what your customers say online and get a pulse on how your brand is perceived. The customizable dashboards and reports give you clear visibility into sentiment trends over time, so you know exactly where your users’ priorities and pain points lie.
More importantly, Chisel’s analysis helps you take prompt action to improve customer experience and ultimately boost loyalty to your brand. If understanding what your users think makes you feel overwhelmed, let Chisel take the hassle out of user sentiment analysis. Sign up for a free trial today to see how their AI-powered solution can deliver unparalleled insights to drive your business forward in 2024 and beyond. Your customers will thank you for it!